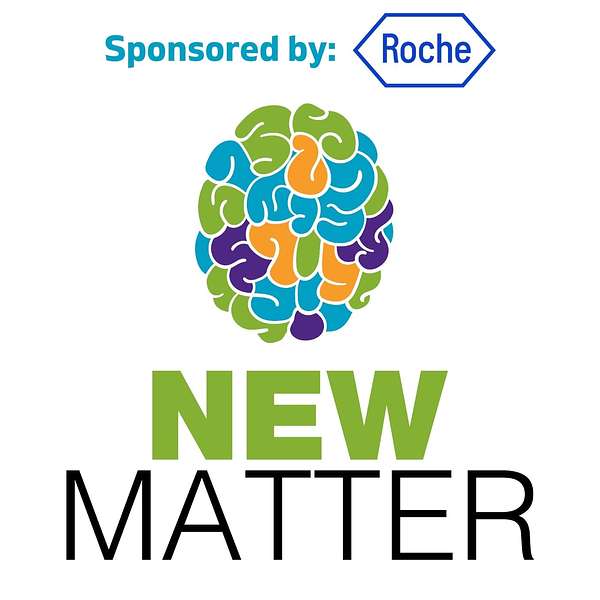
New Matter: Inside the Minds of SLAS Scientists
The official Society for Laboratory Automation and Screening (SLAS) podcast explores advances in automation, cellular imaging, big data and what's coming in the spaces between traditional scientific disciplines. Guests often include members of SLAS along with innovators, leading experts and other members of the global scientific community to highlight technology and even career stories. Episodes are released every week and subscribe to New Matter - available on all podcast players.
New Matter: Inside the Minds of SLAS Scientists
Exploring AI Adoption in Early Drug Discovery with Raminderpal Singh (Sponsored by Roche)
In this episode, we delve into AI adoption in early drug discovery with Raminderpal Singh, Ph.D., founder of HitchhikersAI. Raminderpal shares insights into HitchhikersAI's mission, which focuses on providing a collaborative platform for leveraging AI in drug discovery, emphasizing simple and effective solutions over complexity.
They discuss the distinction between popularization and democratization of AI tools. He highlights the importance of making solutions widely accessible while addressing specific needs in the open-source community.
Learn More
Full Transcript on Buzzsprout
Our Sponsor for this Episode
At Roche Sequencing, we are building on Roche’s legacy of innovation to transform next-generation sequencing and its application. By simplifying workflows and expanding assay menus, we are broadening access to genomic data and lowering barriers to routine use. Our growing suite of products spans the genomics workflow, from sample acquisition and preparation through data analysis and final result, helping you answer important questions in genetics, cancer and beyond.
We’re not just committed to providing you with our broad portfolio of industry-leading sequencing solutions; we’re also dedicated to making sure that you have the scientific support, technical expertise, and guidance that you can depend on. So no matter what your sequencing destination, you can be sure we’re with you every step of the way.
To learn more, visit: sequencing.roche.com
Stay connected with SLAS
About SLAS
SLAS (Society for Laboratory Automation and Screening) is an international professional society of academic, industry and government life sciences researchers and the developers and providers of laboratory automation technology. The SLAS mission is to bring together researchers in academia, industry and government to advance life sciences discovery and technology via education, knowledge exchange and global community building.
Upcoming SLAS Events:
SLAS Europe 2025 Conference and Exhibition
- 20-22 May 2025
- Hamburg, Germany
Hannah Rosen: 00:00:05 Hello everyone, and welcome to New Matter, the SLAS podcast where we interview Life Science luminaries. I'm your host, Hannah Rosen, and today we'll be continuing our series discussing the Lab of the Future. And our guest today is Raminderpal Singh, who's the creator of HitchhikersAI, which is a nonprofit impact community, uh, focusing on accelerating the adoption of AI and ML in early drug discovery globally. Welcome to the podcast Raminderpal.
Raminderpal Singh: 00:00:31 Hey, thank you. Pleasure.
Hannah Rosen: 00:00:32 Oh, our pleasure to have you. So, to start us off, can you just, uh, provide us with a little bit of your professional background?
Raminderpal Singh: 00:00:39 Yeah, sure. So, um, at my PhD actually my studies were in semiconductors, and then I went, so did that in UK, I'm in the UK at the Newcastle University. And then I went over to California, um, worked in, uh, San Jose for about three years. Then I went over to Vermont. Uh, so I was in the semiconductor industry doing this, uh, software side of things. Um, then I went over to Vermont and I worked for IBM, the Semiconductors group. And then I went to New York, um, after that and worked, uh, moved over from a techie to business stuff, um, business development, et cetera, IBM research, and that's where I go into healthcare. And, uh, Watson Genomics specifically, uh, played a leading role, uh, within IBM research, getting that out to the market. Um, then I left, um, came back to the UK about 10 years ago, 9 years ago.
Raminderpal Singh: 00:01:31 Worked as a sales guy. Really dark side stuff at this point. I mean, very, you know, sold my soul. Um, and then it recently, I gave all that up, started a company called Incubate Bio, which is trying to do some very classic, uh, complex, classically complex AI for the life sciences drug discovery. That's, that's happening in the, in the side and, just in the last six months or so, I've got ultra excited about this non-profit or this problem, this, uh, supply chain ecosystem problem in early drug discovery. So I started putting a lot of the time into this HitchhikersAI thing recently. Now for all that, I've done a bunch of standards to body stuff, I've listened a couple of books, I've got a bunch of patents, it's been a lot of fun. And now I'm a point of, you know, trying to culminate that into something useful so that people can actually get some value, uh, back, if that makes sense.
Hannah Rosen: 00:02:28 Yeah, definitely. That's fantastic. It's quite the journey you've been on.
Raminderpal Singh: 00:02:32 <laugh> It's, it, it's quite this, it's, it's more of a rollercoaster, right? <laugh> Six Flags event this is.
Hannah Rosen: 00:02:39 What is hitchhikersai.org?
Raminderpal Singh: 00:02:42 Okay. So, um, hitchhiker comes from Hitchhiker's Guide to the Galaxy, just made that up 'cause big fan, any Americans out there go to look at the link, it's at the bottom of the page. You can go even listen to some of the, uh, BBC radio shows, all that, that, so that's a name. The, the name is just something out of interest and to have some fun. But what's the point? The point is, is that we see this in other industries, AI is a big deal. Lots of companies changing the way they operate. We've known about this for some time. You can even look at the cars, you know, what is the car these days? Where's the car in the next five years? So, what is early drug discovery? And the problem is this early drug discovery hasn't really changed much in 30 or 40 years.
Raminderpal Singh: 00:03:20 You've got some areas like informatics where there's been a big boost of, uh, molecular design. Uh, you've got some areas where there's, there's improved use of machine learning, computational techniques, more data's available. It's all bits and bobs. And I looked at this and, and I was a vendor in this space for many years, uh, while I was doing Watson Genomics, and then here, um, in my Incubate Bio, and I looked and, said like, there's, there's really a systemic problem here. So, I go solve the systemic problem. And it's very niche, don't get me wrong. It's a very niche problem. And it's very, um, uh, very difficult to, to describe people. Like, to talk about, oh, I'm into Alzheimer's disease, or I'm into certain types of cancer, or I'm going to do, you know, large language models and do another ChatGPT.
Raminderpal Singh: 00:04:09 And they, they have these shiny objects. But then you talk about, well, no, I'm trying to solve the actual problem at an ecosystem level and people, very easy to lose people, this is where I'm excited, um, think of it, systems thinking as opposed to, you know, thinking about any units and, uh, shiny objects. And I started talking to some folks and I was very surprised actually, I'm not the only one. And, you know how that is, you look for a bit of confirmation bias, you get your confirmation bias, and you start going on your own Kool-Aid. That's where I am. So, we started creating the, the messaging. We started creating what we're trying to do and think of grassroots people were just, there's about 150, 160 people in and people will just come aboard and say, you know, I wanna do this.
Raminderpal Singh: 00:04:54 Like, I have this box predictor, this, uh, test predictor, um, and we've been working on it for a couple of years and we haven't found a way of getting it out so people can use it, all open source. Cool, bring it along. So, if you go on the website, you like to see a video, the latest on this video, she likes code, it's on CoLab, et cetera. But the problem with all these things is they're very interesting talk about, and they're almost like doing another GitHub, GitHub, uh, software source repo. The problem is, is that if you're gonna make a difference, you have to think about the bench scientist who could be a biologist or chemist. And that's where Hitchhikers is very different. We've, we're myopically focused on changing, improving the workflow that bench scientists have to do in early drug of discovery. And that's why we're not a drug, uh, so not a source depo, we're not a data bank purely, we're not all these different things. Fact, we don't mind if anybody else does all that. What we are is very focused on the bench scientists and making their lives 10x more powerful, cheap, uh, faster, better, and more, uh, creative.
Hannah Rosen: 00:06:02 Hmm, that's so interesting. 'cause yeah, I feel like when you talk about AI and machine learning, you, you first think of the dry lab scientists, you know, the computer scientists, the data researchers. Yeah. And so how, how does, how do the wet lab scientists tie into this?
Raminderpal Singh: 00:06:17 Right. So, so they're the bench scientists I'm talking about, right? And and they're people you've gotta help. If you can't help them, then who are you helping? Uh, we take a typical biotech or we take even a biopharma, you know, their early drug discovery, you know, vertical, what are they chartered to do? They're chartered to get data. That's what they're chartered to do. They're biotech. You're not gonna raise money if you don't have good data. If, if you are in big biopharma, you're not, your program's not gonna progress if you don't have good data. So, you have to get data. But that data's wet lab data, it's not, you know, run a machine learning algorithm and tell me what it says. No, I wanna see the wet lab data that's a bit archaic, don't get me wrong. I think that one in itself will start to change over time, but at the moment, that's where we are.
Raminderpal Singh: 00:06:58 So if you are not helping that person get data, you're not thinking about what the, what the actual, uh, problem to be solved is, job to be done. As they say in business, the jobs to be done. And what you're doing is you're just pushing assets out and say, look, I've got great assets. And it's very hard because it's very easy to be very, to get hyper excited about technology, algorithms, whatever, you know, super computers, very easy to get hyper excited, quantum computers, let's get excited. But at the end of the day, that bench scientist has to get something across the line, has to get dataset, and those people don't like computers very much. They, they'll play with computers, in fact, in the old days, they used to use computers a lot more than they did today. 'cause in the old days before there were lots of computational, uh, sorry, computer science people in the, in the room, they were the people using spreadsheets and they're the people, you know, who were managing the data. But today, all that's being delegated, well not all of it but most have been delegated. So, they actually use computers less than they were using 20 years ago. It was a real weird state of affairs, and therefore they're kind of cautious about all the promises that are coming. And that's a real problem that needs to be solved.
Hannah Rosen: 00:08:14 Interesting. Yeah. So, what, what is your, what is your goal through HitchhikersAI? Is it to increase awareness among bench scientists of the needs, uh, for what their data needs to look like in order for AI and ML to work? Or, you know, how, how are you helping to improve the workflows for bench scientists?
Raminderpal Singh: 00:08:37 Yeah, no, it's a very good question. The, the first thing starts with thinking about their world. So, we're going and pushing a survey out. We're actually pushing a number of surveys out, starting with the pre-survey. Um, and ask them, where is your problem? Where are you stuck? Are you aware of the AI opportunities? Are you having problems adopting them or you just don’t know about them? Or you've just got an area that you think maybe they could help? And you just, where's your awareness? What in, in, in sales language, we call this the buyer journey, where are you on the buyer's journey on this topic? What are you resisting? What are you interested in? What's slowing you down? Pick problems which people are facing. Don't pick problems that you, you believe they're facing. Pick problems that they're saying they're facing. And that's the part of that is looking for patterns in these survey results.
Raminderpal Singh: 00:09:24 Now that you are representing, you are on their side. You, you, you are their power. So, you are gonna help them out with the issues they've brought to the table. And now you address it by saying, okay, does that answer already exist? Who's got the answer? If not, should we write some new codes to simply, you know, address it in some simple and effective way? But then the code doesn't help, 'cause they're not, they're not code users, they're not, they're not software people. Ok so then how we package that code. Can we put some data on that? Can we put some, you know, make it a no code environment? Can we put some learning and some training? Can we put some, you know, even some certification around that? Can we sort advisory services around that?
Raminderpal Singh: 00:10:05 If we can package this sufficiently, it will get them across the line and say, you know what? That's not so hard, I'll have a shot with that. And because it's all open source and because, you know, we're all on the same side of the table as these folks, we’re their pals, then what that means is, is that they don't view it as a vendor buy relationship. They don't view it as a way of trying to take their money. They actually genuinely believe, you know, I, these people are here to help me. And this advocacy is very important to establish. If you're not advocating, if you're not, if they don't believe you are on their side of the table, that you are their advocate, then you're on the other side of the table, and that's a difficult place. There's, there's distance now. So, advocacy and trust are critical in winning the hearts and minds of the bench scientists.
Hannah Rosen: 00:10:51 Yeah, and, and it's so interesting 'cause I remember, you know, even back when I was in grad school and doing bench work, and you really did need to be a jack of all trades, you know, you couldn't just focus on the area of science you were researching. You did have to learn coding, you had to learn all of these different programs. You know, I spent an insane amount of time learning how to use MATLAB and all this stuff. Whereas what I really wanted to be doing was learning the immunohistochemistry techniques so that I could, you know, actually be looking at my tissue samples and instead I had to be learning all of this coding and stuff. And so that was just the way it was back then, as you said. And it's interesting now that the focus that you're talking about is shifting towards where people can become more specialized and actually doing the experiments and not needing to necessarily learn all of these other, you know, coding techniques and principles, which I think back then it was looked at, you're not a real scientist if you're not also doing the coding and the data analysis and all that stuff. Whereas now you're talking about a shift more towards being specialized in your, your area of interest in your lab work. And do you, you wanna make it so that people don't have to worry about learning an entire new skill or technique?
Raminderpal Singh: 00:12:10 That's correct. And, and the problem is that, so that transition's already occurred. And so, but the problem is, is that now if I'm a very specialized immuno-oncology rare disease, wherever I'm going in this and I don't know how to manage data, you know, do data imputations, for example, or, or even find relevant data sets, let alone doing modeling and algorithms, who's gonna do it for me if that person who's not gonna do is, is if those methods, if those people, if those things are not close to me and they're not in that advocate trusted circle, then I probably won't do it. And it creates a massive slowdown in the data crunching that goes on there. So, you're totally right that there needs to be the specialization because things are getting far more complex than they there have been. Um, we can get the specialization, why not, but it has to be done correctly, not just, um, ivory towers where every ivory tower is best in class, but actually as a system, they don't really work that well together.
Hannah Rosen: 00:13:13 Hmm, that's a very good point. Yeah. If you get too siloed, then you, you just don't know what your data you're producing is gonna be used for or, you know, they, it becomes much more difficult to see the full vision, um, and to get anything done, I would imagine.
Raminderpal Singh: 00:13:30 And, and, and what happened is that this, so this is, uh, over the last 5, 10 years, partly because the cost of data generation is so low now, I mean, look at genome sequencing for example, a hundred bucks. I mean, where are we going with that? Um, the problem is, is that companies therefore look at this, and you can see this in big pharma over the last 5, 10 years and they've created big programs. I wanna have a data lake, I'm gonna get a data lake and I'm gonna stick everything, all my company, all my science data into one big massive database. They've gotta access it. Or I'm gonna have a, there's a lot of governments, so, you know, in the UK as well as in US, these big programs, I'm gonna stick data here and have analytics layers. I'm gonna do this, I'm gonna do that.
Raminderpal Singh: 00:14:08 And these are grand design for, uh, solutions. I have a grand design of what needs to happen. I'm gonna make it all happen. And folk keep finding money until the object exists. But think about that bench scientist, the bench scientist doesn't see anything like that. The bench says, I need help. So actually what you want is, you want a, you want a bit of grand design to understand what are the big objects that may need to exist, but your money and your value or, or your progress, your impacts are gonna come when the individuals in those bench sciences are getting things that they can understand, they can absorb, they can play with it. They get, they trust, they trust the person giving it to them. Advocacy's there, they, they can phone somebody up if they dunno how to use it. And many times those people won't exist in the same organization or the same company as them. So they, so you need a place and that's where Hitchhikers continues to focus its mind on.
Hannah Rosen: 00:15:02 Yeah, and 'cause it sounds like, you know, if you are, if you're working at a really large company, you probably do have all of those people on staff who, you know, you've got the bench scientists, you've got the, the data scientists, you've got all of these different people. But if you're working in a much smaller company or a startup, you probably don't have those resources available to you. And so, I would imagine that HitchhikersAI would be of particular use to somebody who's working with a very limited amount of resources available to them.
Raminderpal Singh: 00:15:32 Yeah, you've got, um, there, there's two angles. This one angle you, uh, spawn. Uh, my guess is that up until about raise about 50 or 100 million, company is still at that point or be small to mid-size biotech where they don't have the necessary skills. They might have a bioinformatician here, or they might have a cheminformatician here. They might have some people on staff, but they really don't have the suite that they need. 'cause it's, it's very multidisciplinary doing early job discovery. It's very complex, lots of different pieces running around. You have these pieces, you don't have those pieces. Um, but if you're bigger than that, you can start hiring the whole teams. So, I think you're right on that or you’re right spot. But I, I think the other angle is that even if you're bigger, you'd have a scale problem.
Raminderpal Singh: 00:16:15 So let's say you want to do a, a build versus buy on data. I need to get some data. Should I put my own test or should I buy the data? Now that's different if you are the CEO and then you can sit with your team and have that conversation in the room. Small guys, that's the easiest of that conversation. But if you are a CEO or senior vice president in a large company, you're actually turning around and say, look, I need, I need guidelines for protocols that my, my groups are following, almost like standards, which I know decent standards, which are learning over time. And I need a trusted group to develop those standards. So, the needs for the big guys are there, but they're different types of needs. The needs for the small guys, as you say, are, are the small to medium sized guys are more direct and they're based upon access and understanding of technology.
Hannah Rosen: 00:17:00 Very interesting. Yeah. You know, this comes in, I feel like, you know, what we're talking a lot about is this pipeline essentially between wet labs and dry labs and kind of getting that flow of information going and, you know, can you talk a little bit in your experience, you know, as we've had this just explosion of conversations around AI over the past couple years, and part of that I think is because of, you know, things like ChatGPT, which has just really, you know, increased our awareness of what the capabilities of AI are. But how is that changing the dynamics of this pipeline between wet labs and dry labs?
Raminderpal Singh: 00:17:40 Yeah, so what you are having, I mean that's, that's specific to natural large language models. So, I won't answer, I mean I can, there's a large language model of conversations to be had, but you asked a pipes question. So, a pipe means I have characters in the room doing different jobs and I want them to work very well together. So, they need to be connected. And that connector, that pipe needs to provide, you know, information for one to another observation, uh, request. That's how all systems work. There's not just a network of events. When people turn up and they've got some fancy AI, they don't think about, okay, what does that enable in that network? What they think about is, Hey, I've got some great thing, which is gonna change the paradigm of the way everything's done, right? This is gonna solve it, right?
Raminderpal Singh: 00:18:28 We're done. I've solved it. No body knows what I did, but I solved it, I don't even know why solve it, but I'm gonna call it “it”. Um, and, and that so people aren't positioning and understanding what the values are. So, if you, but if you sit down and you talk to people who do LLMs, which are now a bit more, um, you know, popular meaning that people using them more, they get it. And what you are finding is people say, well, they're good for this and also good for that. Or if we enhance it, we can do it over here. And you're starting where people are starting to see it less as a showboat, more as a, a cog in the system. But, but if you take a cog in the system approach, you need to understand what the other cogs are doing and that that requires multiple different types of skills.
Raminderpal Singh: 00:19:09 And we're still not there yet. So, multidisciplinary teams, even within AI, is a problem. If I'm an LLM guy, I'm not the same, I don't have the same skills as somebody who goes doing random forest, you know, machine learning using scikit, uh, you know, uh, you know, Python libraries, it's not the same skills. I might have done that before, but it's not same skills at this point or that's probably not the same skills as somebody who spends all the time looking at proteomic data and tries to understand the administration issues in proteomics data. They're not the same skills. But that's all, that's all ML. It's all kind of under that banner of dry lab. And so, we need to evolve to the point where the multidisciplinary, uh, dry lab skills are there to put the cogs so that the best use of these technologies, the appropriate use, you know, not the overselling, it almost the underselling use of these technologies is nicely placed.
Raminderpal Singh: 00:20:00 And we want, we wanna force multiplier out this. So if I use four, five cogs in the system, I want, I want a 100X benefit, I don't wanna turn up and say, LM solves it, we're done. I want LLM in here, and a bit of this, bit of that, work out what needs to happen with all the different skills I need to, to make that correct. And then the, the final result is, is a hundred times better than I would've got individually. And, and some of that's really hard. So again, Hitchhikers is very focused on these sort of cultural, uh, tones to make sure we're not missing the point here in what the industry, the ecosystem needs.
Hannah Rosen: 00:20:37 Yeah, I mean I can imagine that does sound very challenging because you have to balance this. We can't expect one person to know everything because there's too, too much. But also, we can't get too siloed because then you'll miss what is out there. And so it sounds like the solution is, uh, just constant communication within these different groups, which I can imagine is really difficult to, to keep that going because it is a lot of work to constantly be going out there and double checking your work and asking other people, you know, is there a better solution? Is there another way to do it? And so, is HitchhikersAI making this easier to kind of get that cross communication going?
Raminderpal Singh: 00:21:19 So the analogy might be, if you have a target, you know, uh, you're doing some early drug screening and you have a target and you've not validated the target and you've got, so for this high risk target and you wanna solve or play with this high risk target, you need a different environment, you would do a different set of activities with that you compared if you'd validated it. So, we're in the unvalidated world of open source where we are sampling, testing, exploring what's, what, what makes sense. But, but, and the difference between us and vendors, 'cause vendors have a role and we want vendors to succeed, is we're all about simple and effective solutions. We're not about advanced and complex solutions, um, which are needed. They are needed. But if you wanna get your feet wet in a, uh, you know, just learn in a kind of exploitive mode, you just want something simple that you can mess with. And so yes, you're right. We're, you, you could argue it's, precompetitive probably not the best word. The best word is probably that we are allowing people to gain the confidence to, to take, uh, to invest in, in, in these complex topics.
Hannah Rosen: 00:22:30 It's interesting, you know, I feel like this kind of ties into these concepts around popularization versus democratization, which is something that you've talked about before around these concepts of AI and ML. So, could you speak a little bit of, you know, what do you mean when you differentiate between popularization versus democratization and how do these each play a role when it comes to AI and drug discovery?
Raminderpal Singh: 00:22:56 Yeah, no, thank you for the question. And I know I'm gonna get all the definitions wrong here, sorry, <laugh>. I'm hoping, hopefully the listeners come back and comment or, what is he on, you know? Um, so, so the way to think about is a lot of stuff, and I'm gonna use democratization as a, um, democratization, is that everybody gets access. That's, we need that, there's no question about that. And no one’s debating this. So, an open-source program, democratization is you're finding those things which, you know, you, which you'd want people to use because they're the, they're, they're hitting the pain points, they're addressing specific needs. And so, if I'm in a democratization game, and we should both have acquired, if I'm in a democratization game, I'm in the business of build, spread the, spread the wealth. If I'm in the business of popularization, I'm in the business of understand what's to build, build it, make sure you got it right and then, and then specifically push those, that short list of objects out for people to go use.
Raminderpal Singh: 00:23:56 And that's, there's a subtle difference between those two. Um, you want things to become popular because they're the right things. That's what the market needs in their early due discovery, you know, bench scientists in their workflow and therefore they're gonna pick 'em up. And so, if you think about metrics, you know, metrics, every organization has their metrics, every project metrics. It's better for some things to be used and impacting and do one or two things than it is to have 20 things, which everybody thinks are great topics, but actually aren't actually touched. So having source code, which is open source, having a program which is really transparent and readily, you know, accessible, is one value proposition, but it's not the big value proposition. The big value proposition is that the bench scientists love it, use it, and you know, want more. And that's what's, why I'm trying to through that, um, different, uh, statements.
Hannah Rosen: 00:24:49 Yeah, that definitely makes sense. 'cause yeah, just because something is accessible to you doesn't mean it's gonna be particularly helpful to you. So yeah, if we just make sure that, you know, we have a code that everyone can use, but it doesn't apply to their research or they don't want to use it, then yeah, it's a lot less helpful. So, I think that yeah, that totally makes sense,
Raminderpal Singh: 00:25:07 Right? And, but it's not only, even if it is helpful, if I'm satisfied that I've now given access, I stop. But what happens if they've not, they haven't got the confidence at that point to actually take the, take the package, and we call them enable packages on the website, I described this as an old funny green picture, um, and it's quite a weird, but the, um, they take, they take these enablement packages and they use them. Now, you know, you're into something. So, it's all, it's also about metrics, need to understand the, what are you here for, what are you actually trying to achieve in that program and stick to it.
Hannah Rosen: 00:25:44 Very interesting. You know, I'm curious, what was it about your experiences and your background that inspired you to create HitchhikersAI to see the need for this in, in our field?
Raminderpal Singh: 00:25:59 Colloquially it's because I'm not young and I wanted to do something which is actually quite fun. You know, I'll tell you this, there's nothing more fun than a nonprofit, 'cause, that's probably a very general statement, but at least my experience so far, um, because you, your your mission vision values, um, where you're trying to go, what you're trying to achieve, you're, you're not tied to the hockey stick, uh, you know, approach, which is expected in for-profits. You know, I'm building an asset, I'm selling the asset, um, I'm making money, companies capital values going up 10 times every week. Um, you're not doing that. You're focusing on a problem you're trying to solve and going after it. And my, I have an engineering background and I don't think that's, I can't shake that off, that's part of the problem. Um, but why this particular problem? I think it's because it intrigues me that in this industry, and I've only been in this industry for about 10 years, 12 years in this industry, people know this exists, but nobody really, the industry is not actually structured to address this.
Raminderpal Singh: 00:26:59 The industry is about big things, big technologies, big drugs, big companies, big money raises. It's all about, you know, it's a very high ultra, uh, IP uh, you know, dominated industry. It's not about, okay, let's connect a few dots and make, make that work for that individual. A lot of people talk about these topics, but you need to go to academia. What are they doing? Because they're doing the same things the biotechs are doing actually, a lot of the professors, the academics, and they're doing a really good job, but, um, they, nobody's thinking about, okay, how do I connect the pieces together and how do I get the bench scientists to a better place? Even if you go to folks who are focused on specific diseases, I'm here to solve Alzheimer's, solve Alzheimer's, it's all the rah rah. But if you think about the, the base, the foundation, the plumbing and the putting the people together those pipes we talked about, it just doesn't have the razmataz. We all like to, you know, talk about all the logos, you know, and, and, and, uh, yeah, captions, and that intrigues me. That intrigues me. Sometimes it's more fun to work on the problem that nobody really cares about, but actually is quite, quite complex. So intellectually it's quite, uh, interesting, um, because then you can, you're not trying to chase the money. You're trying to chase solving the problem.
Hannah Rosen: 00:28:20 Yeah. And, and what do you think about people, 'cause you know, I think that particularly with bench scientists a lot of the time, you know, with the concepts that they understand around AI and they look at it and they say to themselves or to others, you know, AI is, it's amazing what we can do, but it's not quite at the level that we need it to really, you know, be able to use it for drug discovery. You know, it's not at the point now where we can just plug and play and, and come up with these computer generated models of a drug target and go in and test it and it's gonna be perfect. And so, I'm gonna wait until AI becomes more advanced and I can really like, you know, use it in my research in this way. You know, what, what are your thoughts around that perspective and what would you say to those people?
Raminderpal Singh: 00:29:10 Okay, so, so first of all they’re right on the first statement, which is not ready, and use the term plug and play, um, what plug and play? I mean, that's the problem, right? You've got the vendors and the AI people saying plug and play, and you got the bench scientist saying, no, I don't think so. If both of them came down a couple of levels and said, well, it's not plug and play, maybe there's a conversation which could be had on, okay, how can it be used? So definitely the plug and play is part of the problem, that term, which is used very often. Correct? Then, then your, your comment was what, what to say? How to get them, how to get them to socialize? The, the, if you think about electric cars, so what's the problem with electric cars? The problem is if Tesla is the only person producing, and they're not, there are many now, but if we go back a few years, Tesla, Tesla's the only people producing pure electric cars and the other car vendors, GM, Ford, et cetera, Chevrolet, et cetera, turn around and say, we'll wait until the electric cars will work.
Raminderpal Singh: 00:30:04 Or let's say even more, let's talk about self-driving, oh we'll wait until it works. The day it works will be the day you lost the market because you've got 5 to 10 years to catch those guys up, right? And so now the argument will be, well, that's not a problem 'cause there's a whole bunch of vendors who sell all this AI stuff. Um, and I often talk about the analogy between this and semiconductors industry who have been through this same journey 'cause I was part of that journey. And the idea is, well, the vendors will be able to sell us the software. Yes, they will be able to sell you software. But the problem is, is the, the use of this, 'cause it's not plug and play and it'll never be plug and play. This is biology, right? And, and, and here's the way to think about it.
Raminderpal Singh: 00:30:44 If it's plug and play, you bet that the person who's been using it for two or three years has worked out how to put layers on top of it, which have not yet made it to the vendor tools and not also like code layers and thought about methods and interpretations. So, the plug and play part is always the most basic function you can get, but your competitors will already have a learning curve on using these things in advanced workflows. So, you can't wait, you can't wait. This, you can't wait for the electric car, you can't wait for self-driving car, couldn't wait in the semiconductors industry for the use of the tools. And you can't wait here. What you have to do though, is to go on a path which is sensible. So, forget, take the hype down a few levels, think about bits and bobs which would help, think about pain points, which, where it would make a demonstrative impact, a significant impact, invest and toy in those areas and go through it. But again, vendors don't like those arrangements, they're there to take your money. So again, Hitchhikers is trying to have that middle ground to help you do that as a, as a bench scientist.
Hannah Rosen: 00:31:45 What do you have as, you know, for HitchhikersAI, what are some of your short-term plans, uh, for the website? And then what's your, what's your long-term vision?
Raminderpal Singh: 00:31:55 Okay, so, um, long-term vision is to rule the world <laugh>. So, let’s take that off the table, sure, let's start, let's start with short term plans. So, first of all, building a nonprofit, building a community, super exciting, but also, creativity every day. So, I have to get HitchhikersAI, the team who would be there, has to get themselves to the point of, you know, there is a, uh, there is a significant value proposition, which is, let's say a directed value proposition, not significant. When it’s significant, there is a pulse, it has to be directed. So, for example, I was talking to one of our steering committee members today, and he was saying, look, one big problem, thinking about the big topics, less is more. Do less things, but do big things. One big problem maybe to think about is the, in, uh, cheminformatics, uh, you know, AstraZeneca have, um, open-source tool called Reinvent, but Reinvent is very complex for bench scientists to use.
Raminderpal Singh: 00:32:51 And it's very important, this is the protein folding, uh, uh, the compound design phase of things. Maybe we can work out rappers to make Reinvent usable. Uh, another thing is Broad, um, with, uh, Sanger have the dev map database and they've got wonderful layers of how to access all those cell line data. That's really well done. But there's more that can be done in terms of making that much more accessible to bench scientists. So, find those big topics, which are big, 'cause they, they lead to a lot of productivity, a lot of value from bench side. Get a short list of them, double down on them and show progress on them. That's the short term. In the long term, one has to think about, fundamentally, what's the influence that we're trying to, uh, we're trying to, uh, peak at? What, what at what point, because these things, like, there's a saying that companies have a, a lifetime.
Raminderpal Singh: 00:33:38 They think they last forever. Like, uh, this company's 100 years old, this company's 3000 years old, but companies don't last forever. They go through a cycle and then they become irrelevant. Um, so Hitchhikers will have a, HitchhikersAI will have a lifetime, maybe it's 5 years, maybe it's 10 years, but it, it may not need to go long with that because AI will be, it'll be popularized at some point. It'll be there. You won’t, this distant middle ground, this, these pipes will be happening by themselves. So, the long term is to find out, what's that culmination point where AI has done its job in that 5 to 10 year period. And to make sure people understand that, that we're not here for, we're not existing for the purpose of existing, we're purposed to create value. And then once that value cycle was reached, good, we'll close. Not a problem.
Hannah Rosen: 00:34:22 Wow. I love that. That's such a unique vision. And I guess comes back to what you're saying of, you can have, like, the nonprofit being fun 'cause yeah, you can just come in, serve your purpose, and you don't have to worry about that bottom line. And you can really focus on the value and the overarching goals, which I think is, is refreshing and fantastic.
Raminderpal Singh: 00:34:39 Well, it's also fantastic that we, you know, we've got great collaborators and partners, don't get me wrong. I mean, we're talking, right? The SLAS guys, I was there last month, um, at your, you, you, you know, your big, uh, events at the Boston Convention Center, SLAS2024. I mean, these are fun events, you know, in the UK, ELRIG, which is, you know, kind of a sister, a smaller, a sister organization to SLAS, uh, you know, very supportive. In fact, we launched in October at the ELRIG, um, annual drug discovery event in Liverpool. Uh, where, you know, I had a, had a presentation. My point is, is that if you, if you, if you gang up with the right characters, like-minded people, organizations, it's, such a multiplier comes out and you, you get to do a lot less “coding”, you get a lot more value creation by just putting a few pipes together and making sure the pipes work for the bench scientists, once enough pipes are there, the industry will self, self-create pipes.
Raminderpal Singh: 00:35:38 And, and the key is not to be, what's the right word? Not to be dogmatic, not to be, you know, on a, on a trip, which is all about we need to exist, we need to do the next thing, we need to explain what the next year's value proposition, no, no, it, it's gonna, it's gonna go through its thing and people gonna learn. And there's one more point. People who participate must win from this. There's 150 people I mentioned earlier. Every single one of them must have a real win from it. They're meeting people, they're learning, they're writing code, they're getting some, you know, awareness, people learning about them. Um, and they get this brand, you know, uh, projection. Everybody must learn. The vendors must win. The, the, the people providing data, public data must win. The collaborators like SLAS and ELRIG, they must win. Big pharma must win. And, and, and then you even, you create positive loop when you, when you have that kind of, uh, mindset. And so, the, the way I call this is like. a collaboration first ethos; lead with collaboration. Uh, don't do it yourself if you don't have to, but think about the problem, which is those bench scientists just stay myopic on the problem.
Hannah Rosen: 00:36:45 So if there's people out there who are listening, who are really engaged with this idea and they want to become involved, you know, what, what can people do to, to start participating with HitchhikersAI?
Raminderpal Singh: 00:36:56 So, it's very lightweight, very light touch. I mean, it's really easy. Um, nobody signs any piece of paper. Make that easy. Um, if you go on the website, there's a, um, join us page, just, they'll submit an email to me, I’ll add you to the list, you’ll get a, not a newsletter, I try to stay away from formal, uh, mechanisms, but every so often I shoot out an email to the membership, uh, this is what we're doing, you know, can you help out with this and that. We have a Slack channel, not very, not used that much, but we have one. Uh, we meet once a month. Uh, and you know, a meeting invite goes out, you can dial in, uh, which is kind of fun, is good conversation. The most important thing, however, is that it's a way of you seeing what's going on and saying, you know what, I like this topic.
Raminderpal Singh: 00:37:39 Even if it's a new topic, I like this topic, I wanna have a shot of trying to talk about this topic or, or do something in this topic or connect to people in this topic. Um, that kind of friendly environment, it's, it's very, uh, uh, I think it's rare. Um, it's, it's rare to have that because we're all very stressed and we're all very, all trying to, you know, get our companies, our organizations across the line. So, we're here to be, we're here to have folks, light touch, join, and then whatever works for them, that works for us.
Hannah Rosen: 00:38:15 And you had mentioned earlier that one of your big directives is sending out surveys, uh, and everything. And we, we, I know that you have a survey that you just recently came out with that we are gonna have linked to in the show notes here. Could you talk a little bit about what, what that goal of that survey is and who should be filling out that survey?
Raminderpal Singh: 00:38:31 Yes, absolutely. So, anybody who finds this conversation interesting, doesn't matter who you are, even yourself, doesn't matter who you are, if you're finding this conversation interesting from any angle should be filling out, it's four questions, five questions. And it's not meant to be a, it's not meant to find a specific biological or, or chemical or scientific insights. It's meant to be a high-level flyover of what people are thinking, what they're seeing in terms of the challenges of, you know, AI adoption in early drug discovery. That's our thing, early drug discovery. And with it, but it doesn't matter, you may be a bench scientist, you may not be, you may be a vendor, you may be a coder, you may be a, a postdoc, you may be a PhD or master's student. That's all good. The, the diversity of information, there's a, there's a theory which says that it's not about the sizes of the data you collect, it's about the, the richness of the diverse nature of the data you collect. So, you might be a CEO, you may be a VP, it, it may be the CEO of SLAS, Vicki, right? SSo,my point is this, please go to the website. Uh, there's a link there, uh, which you guys are gonna provide. It'll take you five minutes max, push it out and, uh, you know, we would love to get your thoughts. Five, four or five questions. That's all. It's, it's a Google form. It's a simple Google form. Yeah.
Hannah Rosen: 00:39:54 Quick and easy.
Raminderpal Singh: 00:39:55 Yeah.
Hannah Rosen: 00:39:56 And then for next steps, you know, we at SLAS love what you're doing at HitchhikersAI and we're so excited and happy to be involved. And so, I know, you know, this podcast is not the end of our relationship. So, I know you also have a, uh, Technology Idea Exchange that is coming up with SLAS as well. Could you talk a little bit about that?
Raminderpal Singh: 00:40:15 Oh yeah. No, this is fun. This is all fun. This, this is what, this is why collaborations matter. Um, so listen, a big thank you to you guys and to the team. Um, so at, at, at the exchange, you know, I, there's a conversation which needs to continue here and people have the opportunity to be part of the conversation. So, exploration, discovery, what are the topics, I mean, I have several topics which I'll be mentioning. Um, but they're not the right, maybe the right topics. You know, this whole notion of where should we go after, what are the problems, you know, what do the people feel that needs to happen or, or what are their comments on my list? And that discussion, that exploration discovery discussion is, is what we'll be achieving there. And it'll be a lot of fun. Um, because again, I'm, my style isn’t to turn up with 20 slides and say, Hey, I've got the answer. Actually, I don't have the answer. So, we'll start there. My style is to say, look, there's a lot of people who have been talking about this and I kind of gathered a bunch of information, here's what I'm hearing. So, it's not me. Here's what I'm hearing. And let's have a conversation and let's learn and improve it at that exchange meeting.
Hannah Rosen: 00:41:22 And I believe that, that, that live event is currently scheduled to take place on April 18th at 11 am central daylight time. Um, and if individuals aren't able to attend it live, we will also have a recording of the session available on demand in our learning management system APPLIED. So just in case you're listening to this after the fact, but you're still really interested in that, that content, that Technology Idea Exchange will be available, um, to access from our members, uh, through that learning management system APPLIED that we have. So, um, really excited about it. And Raminderpal, thank you so much for joining us today. It's been a really interesting conversation and we're really excited at SLAS too, to continue working with you and HitchhikersAI and to see where this journey goes.
Raminderpal Singh: 00:42:03 Thank you. It's lovely. Appreciate it.