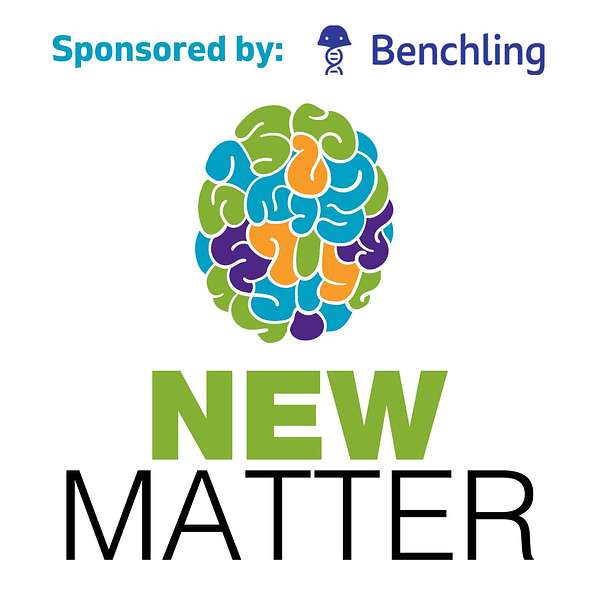
New Matter: Inside the Minds of SLAS Scientists
The official Society for Laboratory Automation and Screening (SLAS) podcast explores advances in automation, cellular imaging, big data and what's coming in the spaces between traditional scientific disciplines. Guests often include members of SLAS along with innovators, leading experts and other members of the global scientific community to highlight technology and even career stories. Episodes are released every week and subscribe to New Matter - available on all podcast players.
New Matter: Inside the Minds of SLAS Scientists
Bridging the Gap in Data Standardization with Glenwood Barbee (Sponsored by Benchling)
In this episode, Hannah Rosen speaks with Glenwood Barbee, M.B.A., Product Marketer at Benchling. Glenwood discusses Benchling Connect – a platform designed to accelerate connectivity and automation in laboratory settings.
Glenwood highlights Benchling's mission to revolutionize biotechnology by bringing modern software to modern science.
The conversation delves into the challenges of data standardization in life sciences. Glenwood outlines customers' primary pain points:
- Disconnected instruments
- Inaccessible data due to proprietary formats
- Fragmented analytics leading to data lineage issues
Overall, the episode sheds light on the critical role of data standardization and automation in driving scientific progress, with Benchling at the forefront of innovation in the life sciences industry.
Full Transcript Available on Buzzsprout
Our Sponsor for this Episode
Benchling creates the software that powers the biotechnology industry. More than 200,000 scientists at over 1,200 companies globally — from cutting-edge start-ups to 20+ of the 50 largest global biopharma — rely on the Benchling R&D Cloud as their central source of truth for scientific data, analysis, and collaboration. Benchling is on a mission to accelerate scientific progress through advanced software that's purpose-built for biology.
To learn more, visit: Benchling.com
Stay connected with SLAS
About SLAS
SLAS (Society for Laboratory Automation and Screening) is an international professional society of academic, industry and government life sciences researchers and the developers and providers of laboratory automation technology. The SLAS mission is to bring together researchers in academia, industry and government to advance life sciences discovery and technology via education, knowledge exchange and global community building.
Upcoming SLAS Events:
SLAS Europe 2025 Conference and Exhibition
- 20-22 May 2025
- Hamburg, Germany
Hannah Rosen: 00:00:05 Hello everyone, and welcome to New Matter, the SLAS podcast, where we interview Life Science luminaries. I'm your host Hannah Rosen, and today we are joined by Glenwood Barbee, product marketer for automation and analytics at Benching. Glenwood is here to talk to us about Benchling Connect, a platform designed to accelerate connectivity and automation in the lab. Welcome to the podcast.
Glenwood Barbee: 00:00:26 Thanks for having me, Hannah. I'm excited to be here.
Hannah Rosen: 00:00:29 Oh, it's our pleasure. So Glenwood, could you start us off with just kind of giving us a brief description of your professional background?
Glenwood Barbee: 00:00:37 Uh, yes. Um, and also, uh, excited to join, uh, since we exhibited last week at SLAS, and, uh, just kudos to you and your team. We, it was an excellent show, so we had tons of great conversation. Uh, quick background on me is that I'm a life sciences management consultant, turned product marketer. Uh, started out working for Deloitte with Fortune 100 Healthcare and life sciences companies, and found myself most drawn to the marketing strategy work. So decided to go to, uh, business school at Stanford and pivot into product marketing. And I've spent the last decade helping mission-driven startups across industries, launch disruptive products, including in Healthtech at Castlight Health, uh, FinTech at Affirm, transportation at Lyft, and productivity at Clockwise. And here at Benchling where I've been, uh, for just over a year, my focus has been on supporting the go-to market of our automation analytics products.
Hannah Rosen: 00:01:26 Great. Well, I'm so glad to hear that you guys had a good experience at SLAS2024. We had a, we had a great time at Boston and I'm glad that you guys did too.
Glenwood Barbee: 00:01:33 Yeah, it was great. It was my, uh, first time out at SLAS, so lots of energy and, and great conversation.
Hannah Rosen: 00:01:39 Oh, nice, nice. Yeah, it was a, it was a great conference this year. We had so, so much fun. Um, so can you tell us a little bit about what is Benchling's overall goal or mission?
Glenwood Barbee: 00:01:50 Yeah, uh, happy to. So Benchling's mission is to unlock the power of biotechnology with the goal of bringing modern software to modern science. Um, so for some of your listeners who may not be as familiar, I can provide a quick snapshot of, uh, what we do, uh, and why bringing modern science software to modern science is our goal. So, the company was founded in 2012 by two MIT students, uh, Ashu and Saji who worked at the bench and were shocked by the tool scientists were using, uh, tools that they described as equivalent to stone age technology, uh, when much of the rest of the world was benefiting from modern digital software. So, name your favorite, uh, app, for example. Um, they saw instances and we've seen instances where scientists are using a multitude of Excel spreadsheets and manual analysis to address software gaps. So that's, uh, what we mean when we say stone age technology. And today, just for context, we serve over 1200 customers and 200,000 scientists globally have over 800 employees across the US in Europe. And one thing I like to note is that over 200 of our team members have scientific backgrounds, PhDs and advanced science, science degrees, and sit on our customer success and services teams and other teams. So really critical for us that we stay close to the science. We have experts in the science who are working hand in hand with our customers.
Hannah Rosen: 00:03:04 Yeah. So, I understand that some of what you guys are focusing on is these issues that we are seeing a lot with data standardization in the life sciences. So, can you describe to us, uh, what are some of the current issues that you've been encountering with your customers around data standardization?
Glenwood Barbee: 00:03:23 Definitely, and that's part of the, uh, inspiration, uh, behind our product vision around automation, analytics and connect. And to start off, we'd love to share a few stats, uh, that really paint the picture from our perspective. So, number one, it's just this macro trend of life sciences data exploding and that the fact that the pain of dealing with this data is getting worse. And we actually ran a study, um, last year, uh, and produced a report called the State of Tech and Biopharma Report, uh, where we surveyed hundreds of professionals in the industry. One of the findings was that R&D data is expected to double in the next year. And so that, that's made a few things clear from our perspective that the growth of data is driven by instruments and high throughput workflows. What's also interesting is like the instruments to people ratio can be as high as one-to-one in labs.
Glenwood Barbee: 00:04:08 And we also found through our survey that 60% of customers connect less than half of their lab instrument. So, it's not only about connecting instrument data, there needs to be the context there. So, it's easy to understand, leverage, reuse, and doing that at scale and alignment with our principles is really hard. And so, with those stats, that really leads into what we see as the three primary pain points our customers are, are facing around data, generally data standardization, writ large, et cetera. The first is disconnected instruments because the instruments are not connected, 60% of customers connect less and then have their instruments transferring the data as manual and spreadsheets over USB drive, which is super time consuming and error prone, which leads to the second problem. If your instruments aren't connected, then the data is inaccessible. And so that's exacerbated by the fact that instrument vendors tend to put their data into proprietary format.
Glenwood Barbee: 00:04:57 So you have tons of instruments all in different formats. On top of that, scientists will often export their data in different ways. CSVs text files, I'm sure you're familiar, and other folks you have talked to are familiar with that rainbow of data file formats. And so, you can think about this problem at scale as like all this data in different formats, in different shapes, and it's really just a nightmare for anyone to search, browse, and understand that data. And then last but not least is this issue of, or this pain point of fragmented analytics. So, scientists like to use the greatest and nice tools for the research, which is great, but the problem is when you copy that data from one tool to another, you lose the data lineage. So, one of the classic examples is, say a scientist downstream wants a file on IND, that data and chart that got generated in PowerPoint or a program review looks great, but then that scientist has no idea where the data was sourced from. If they can trust that data, how that data got transformed over time. So, it creates a huge issue, which is not just matter of time, it's also a matter of money, it's a matter of inhibiting or blocking science and preventing people from reaching their milestones. So that's, uh, that's how we frame the, the problem around those three pain points. Thinking about some of the, like the data we're seeing.
Hannah Rosen: 00:06:09 It's so interesting. You know, just as you were talking about that, I was thinking about, you know, having just come from the SLAS international conference and thinking about all the amazing automation equipment that we were seeing on the exhibition floor, and just, you look at these pieces of, of automated equipment and it looks like that must be, that's the challenge, right? Is to develop this hardware that is like all these complex moving parts and all these, but the more we have these sort of conversations, it seems like the challenges really with the data now that, you know, we, we've gotten the hardware and we seem to have neglected the data as a result. Do you have any insight as to, you know, why is it that we're able to create all of these amazing pieces of laboratory automation equipment and yet the data, which should be seems like the simple bit is proving such a challenge for us?
Glenwood Barbee: 00:06:57 Yeah, that's a, a great question. And I also, uh, it's just a crazy juxtaposition where you're, I was at SLAS last week and you're seeing all these automated machines and complex scientific instruments, and then in our conversations with customers or other folks attending the show at the booth, you ask 'em, what's, what's their, what's your biggest problem? It's like, oh, managing these Excel spreadsheets. So, I think partially it's the fragmentation, right? So, I think there's, there hasn't been alignment around a standard is another one. And then I think generally, and again, I think there'll be different perspectives, it's just general inertia, right? So, I think people are used to doing things a certain way. Um, in the same way I think, uh, we would say like moving from paper and pen, uh, lab notebook to an ELN is a transition, I think it's the same with data. And so, I think also it's a hard problem to solve and, and no one's really solved it well or hit the bullseye or, uh, provided that silver bullet, um, in the market. So, I think that's where you, you see that, uh, versus the sophistication you see on the instrument side with the, with the actual hardware.
Hannah Rosen: 00:08:07 Earlier you kind of mentioned this idea of wanting to keep the data, uh, “FAIR”. Um, and that's something that is becoming more and more important to a number of researchers. So, can you kind of talk a little bit about how is all of these, those problems that you mentioned with having all these differing data standards, how does that impede a researcher's ability to produce FAIR data?
Glenwood Barbee: 00:08:30 Yeah, I think the simple answer is it literally slows down the process because achieving FAIR data, I'll, uh, if I could use that term, uh, hinges on operating off the same standard. And so the, the analog there, I know we, we were talking about analog and digital, but like the, the analog there is really, if you look at other industries and their transformations into digital world, if you think about telecommunications, if you think about banking, you see a similar pattern where standardization is often a key driver. You think about 5G or, or name your favorite standard from another industry. We've approached it at Benchling with a two-prong strategy. So, first the goal is to make Benchling natively compatible with an industry standard and the case of FAIR data, we decided to start by using the Allotrope Simple model, which is governed by the Allotrope Foundation, which some folks are familiar with.
Glenwood Barbee: 00:09:19 And it's a, a leading standard data standard out there. And then second is taking that one step further to accelerate standardization by taking an open source approach. So instead of a closed door approach, we're building an open source repository of data converters to accelerate adoption of, uh, these data standards. And we have published an open source library of data converters for instrument data allotropy. And it's notable for, uh, some several reasons. And one is the first open source data conversion code, uh, repository by a major vendor. As I mentioned, it was developed in collaboration with allotrope and its pharma partners, right? So, with industry leaders, folks pushing the industry. And then third, it provides full visibility, access and rights to adopt or adapt the code for customers own use cases. And the last point I make is contributions are welcome. So, it's on GitHub, if you go to github.com/benchling-open-source/allotropy, you can contribute and see the Read me and, and the code live out in the open.
Hannah Rosen: 00:10:21 That’s pretty unique. Do you ever, you know, as a business, do you ever get pushback for having your code be open source or do you get any concerns from anybody about that?
Glenwood Barbee: 00:10:32 Uh, concerns from customers specifically?
Hannah Rosen: 00:10:34 For customers or from like investors or, you know, employees? Is anybody ever, you know, wondering are we shooting ourselves in the foot by having this as open source?
Glenwood Barbee: 00:10:44 Yeah, that's a great question. I, um, would answer it in a couple of ways. Like, I think for us to see true innovation in the space like, you have to take a step forward there. And so that is, uh, in service of a, of a greater good, of better data. So, I think number one, I think people will see that. And number two, right, from just a pure security perspective, right, it's open source data converters, but we, we have done that, uh, thinking about that from a product security perspective, from an IT perspective so that customers don't have concerns around that. But actually we've seen lots of great buzz around that when we announced it last year. And, uh, people are interested in contributing and, and like the idea of that, right? Given all the reasons we just discussed for why, uh, data is in the complicated state that it's in at the moment when it comes to instruments.
Hannah Rosen: 00:11:38 And I mean, it seems like a great system 'cause you get real time feedback from your customers about, you know, what's working, what's not working and, and potential fixes that they can recommend. I mean, it seems like a, a great solution.
Glenwood Barbee: 00:11:50 Yeah. And I think we're continuing to build it out or continuing to incorporate feedback to make it even better. And I think a lot of the curiosity and questions we've gotten have helped us there. So, that's really part of the, uh, thinking behind it, right? It's like, it's a place where people can communicate and collaborate and share their best practices to accelerate data standardization.
Hannah Rosen: 00:12:11 Yeah, that's great. Can you just kind of, now that you know we're talking about it, can you describe this new Benchling Connect platform, what it is, what it does, how you came up with it?
Glenwood Barbee: 00:12:21 Yeah, the, the quick summary is that, uh, with Benchling Connect companies can increase their R&D throughput and drive better decisions with powerful instrument connectivity and more accurate end-to-end scientific data management. And we see it as a flexible platform built using this unique open source approach. Uh, we just talked about in support of FAIR best practices, and I'm a marketer at heart, so, but this isn't, uh, just marketing language. We're really helping customers speed up their science. So, for example, instead of it taking weeks or months for a customer to integrate an instrument to software, often by using middleware or some patchwork of other homegrown solutions or, or other tools, uh, Connect is helping customers connect their instrument directly to Benchling, to central source of truth in hours. And we've actually been able to deploy, uh, SoftMax Pro, a very popular plate reader software, a data connector for that at a mid-size biopharma customer this fast. And we've seen other results and signals that there's like meaningful significant increases in speed, uh, and connecting instruments.
Hannah Rosen: 00:13:23 So getting down to like, the actual brass tacks of what it can do. So, does this, is this something that, like, if you had, you know, two pieces of equipment from two different vendors, is this Benchling Connect gonna enable those pieces of equipment to, to talk to each other essentially?
Glenwood Barbee: 00:13:38 Yeah, so there's a couple ways, uh, we think about this. So, we both will offer out of the box data connectors or instruments specifically, that can be either an API based connection directly to an instrument software or it can be file based. Um, one of the powerful things about Connect is that if you have an instrument file on a lab PC in the lab, we are able with Connect to transmit that file, pull that file off the PC and push and pull that between Benchling, right? And specifically, uh, one of the cool things too is it's, we have both out of the box data connectors, which do that and standardize the data, um, automatically against, uh, the allotrope simple model. But you all, we also have built-in data tools. So you could actually pull a file from another instrument that maybe doesn't have an out of the box data connector and transform that file eventually into the format that you want and configure it in a way where you don't have to go through all of those manual transformations, all of the exporting and all of the like, transfer of data from, from different machines.
Glenwood Barbee: 00:14:40 And we also can work too, based on we're data strategy agnostic. So, if there are other tools in a customer's ecosystem, uh, we'll come in and be consultative and, and provide a solution of like, some customers are more developer forward and they want to use more API scripting, there's a pathway for that. There's some customers, again, who want a more out of the box solution and we can, we can direct them. Part of the power of Connect is its flexibility.
Hannah Rosen: 00:15:07 So would it be accurate to describe Connect as sort of like a translator where it's taking all these different languages, so to speak, and being able to kind of translate them all into one language so that everything can talk to each other?
Glenwood Barbee: 00:15:19 Yeah, that's a, that's an apt analogy. So, we can either do that out of the box we've chosen and, and, uh, put a stake in the ground around supporting allotrope, but can also translate it into the language and the format that fits that customer's use case or needs, uh, using metadata tools, um, provided by Connect.
Hannah Rosen: 00:15:37 Oh, that's great. Yeah. Flexibility indeed. I like that. That sounds very, very handy. Very useful. What inspired you guys to develop the Benchling Connect?
Glenwood Barbee: 00:15:46 Really these instrument connectivity and data management challenges, having developed Benchling and matured the product, the team kept coming across these things. So that's part of the inspiration. And, you know, everybody has, I shared a couple examples, but there's, there's many more. Given the maturity of where Benchling was as a platform, we felt we could offer the customer then the market a more holistic and innovative product focused around native instrument connectivity, right, built in scientific context and metadata. One of the overall values of Connect or the value it provides is the ability to connect to that scientific metadata, right? So, you can keep that lineage that there is that, uh, throughline from the bench science work also that this open source industry standard and allotropy and these data converters are really important. So there's, that doesn't require vendor lockin, it prevents vendor lock in and provides future proofing.
Glenwood Barbee: 00:16:36 So things change, vendors may change, right? Like the, the lab setup may change or not locked into a specific platform or, or middleware. And then the flexibility, I, I mentioned this in a little bit in the other answer is to support all types of data strategies. So, we're data agnostic, right? We provide, we can provide a data warehouse to customers. We know that some, uh, customers come with their data lakes. Some are more sophisticated, some are further along in their, in their data journey than others. So, um, we really wanted to provide that flexibility to support data, different data ecosystems and, and, uh, use cases for customers.
Hannah Rosen: 00:17:11 Are there any particular vendors that you work with directly in order to get these connections going? Or are you guys pretty like you, you can work kind of independently to get this working?
Glenwood Barbee: 00:17:22 Yeah, that's a great, a great question. I, I would answer by saying part of the value too is the, the team that we bring to this. So, we set this up and in a way that we can work with our customer success and professional services teams to help diagnose and, and figure out the best solution for customers, both from an out of the box perspective. So, um, we're vendor agnostic, um, from that perspective. But, um, working, uh, with partners for example, you know, we just launched a partnership with Clustermarket, which is equipment and laboratory scheduling software. So, we're, we also look for partnerships within the ecosystem that are adjacent or support connect.
Hannah Rosen: 00:17:59 What are some of the challenges that you guys faced while developing Connect?
Glenwood Barbee: 00:18:02 Yeah, it is a, it is a challenging nuance space and problem. And I think a lot of the challenges are similar to kind of any company who's, who's built or is building a data intensive products. So, a couple things come to mind. SSo,lots of complicated and nuanced data modeling and engineering is involved both like modeling, uh, sciences complicated. I don't, I think that's a pretty, um, obvious point that everybody who listens is aware of. And so, bringing that into the product, there's a lot involved there. I would say second, identifying the appropriate use cases to build requires a significant amount of thought given, uh, in many cases, every customer's situation is, is different. There are different challenges. There's such fragmentation in the instrument space and what types of data are being used for what types of science. And there's, you know, it's so specific and unique to both companies in different areas.
Glenwood Barbee: 00:18:55 Also, we found that no one in the industry really doing this well, as I mentioned, or has that silver bullet solution. So, there isn't a pristine or exact model to follow. So, uh, using another metaphor, we're kind of really bush whacking that path, right? We're carving that, carving that path, the approach we're taking. So, all challenges like that have made the product better. I think we've, uh, we're taking an approach that allows us to, to work through those things, but data is data. We wouldn't be, uh, chatting if data wasn't, uh, difficult and, uh, complicated to deal with, um, sometimes.
Hannah Rosen: 00:19:30 Yeah, absolutely. It does seem like more and more this is the issue that we're hearing everybody's saying is the data standardization, what are we gonna do about it? So, I you guys are certainly timely with being on the market. People want it, people need it.
Glenwood Barbee: 00:19:44 Yeah, and I think that's, uh, to your point, it's, it's, it's a, it's such a clear need even from a first principle's perspective, like, most of the data generated from experience, not all of it in all cases, but a huge, um, um, majority of it or a vast majority of it, it's coming from instruments, right? So, it's really critical. So, we're happy to, to work on this problem and help and help address the need.
Hannah Rosen: 00:20:06 You'd mentioned, you know, there's so much diversity in the type of research and the type of equipment and instrumentation that people are using in the life sciences and, and, and laboratory research in general. So, are there any, you know, specific types of research or instrumentation that Connect works the best with?
Glenwood Barbee: 00:20:25 Yeah, it, it comes back to the flexibility point. The beauty of Connect is it's designed to work with, uh, with a wide variety of research and lab instrumentation. And maybe I'll put a finer point on those two ways to connect lab instruments. So, there's outta the box data connectors standardized, uh, to the outro simple model. So, we currently offer 10 plus around 14 out the box connectors across nine instrument software categories. And then those include common code, uh, instrument software like SoftMax, Pro, Want Studio, et cetera. But we also were thoughtful there about like, what are the most commonly used instruments, what are market leading instrument software and, uh, work to prioritize that on our roadmap. And then the second piece, which is super powerful when combined with out the box data connectors is this idea that we, um, can take a universal data connector, what I mentioned a little earlier about being able to pull a file and push and pull that between Benchling and lab PC and what we're calling our point and click data parsing tools.
Glenwood Barbee: 00:21:21 So that allows folks to transmit their flat files between their lab, PC and Benchling, and then we can use our data tools to transform and parse that data within Benchling. So specifically let's say you ingest a file from SoftMax Pro. In Benchling, you can literally say, I want to run these transformations of the, on the data and, and pointing, click those things and actually preview what that data looks like, so you don't have to toggle back and forth between the files. Um, and then you can save this run or this configuration. And so every time you pull the data off your lab PC or local server or machine into Benchling, it'll actually be transformed into the format that you like so that you have it in Benchling, it's associated with that metadata, then you can turn it into a dataset, push it to an analytics tool, and actually, um, have that data in a form that's, uh, that's structured and usable versus spending hours trying to fiddle with the lookups or conversions or other file formats, those sorts of things.
Hannah Rosen: 00:22:21 Um, what are the current limitations of the Connect platform?
Glenwood Barbee: 00:22:25 Yeah, I would say the, the main one is just due to the fragmentation, the instrument market and the long tail of instruments and software or even hardware in the lab. There may be some edge use cases that are harder for Connect to support than others. That's, that's why we've invested in data tools and continue to invest in data tools. And also I, uh, to note that we have an API and a developer platform, so we offer multiple avenues, um, to support those. There's cases, but there's some cases where it's harder. There may be some obscure instrumentation or some very super specific edge cases, which is maybe necessary for a very unique type of science that's happening where it's harder support, where it's just not like as straightforward as a, as a plate reader use case.
Hannah Rosen: 00:23:09 Do you have any plans for future development, um, with either Connect or within this area of data standardization?
Glenwood Barbee: 00:23:15 Uh, yes. Yeah, we're really excited about the roadmap and I would say there's two different pathways that we're excited to expand Connect along. So, one is just the connectivity roadmap, so both on the out of the box data connector fronts and the data tools front so that we continue to support more and more instruments and instrument use cases. And then we're releasing a whole set of features this year that enable customers to catalog and manage their instrument, other R&D data, both raw and unstructured. So, part of the power of Connect from a data lineage perspective is not just capturing the structured data, right? Um, it's capturing the raw data and, and the files and Benchling. And so, a couple examples of that is like, once you've ingested and processed your data or transformed it, you'll be able to manage that data directly in Benchling.
Glenwood Barbee: 00:24:02 So features like the ability to browse and search data, preview the data and its associated metadata, take actions on it, analyze it, download it, archive it, and then point and click analysis and dataset creation. So those are some of the things that we're thinking about. We've already started to, to roll out some of those, some of those features and beta, and I would just say too, our roadmap's really in service of product innovations that enable fairer and more AI ready data so that customers and um, and folks in the industry can really unlock the power of it. So for example, one of the exciting things on our roadmap that we're beta testing and developing and our features that allow customers to generate charts and reports and Benchling using LLM, so you could type in a prompt and then generate a report that may take you hours to put together, but that's all hinges and comes back to having harmonized data that's structured in the right format. Uh, so that AI that data is ready for that optimization to automatically generate report. So, lots of exciting stuff ahead for, for Connect, um, and eventually in general this year from a, from a roadmap development perspective.
Hannah Rosen: 00:25:09 That really sounds awesome and very exciting. I'm sure that there's a lot of people out there listening who will really look forward to utilizing some of those features. So, Glenwood, thank you so much for joining us today. This has been a really interesting conversation and thank you to Benchling for, you know, attending SLAS2024 and we really look forward to seeing what you guys come up with this year and seeing you guys next year at SLAS2025.
Glenwood Barbee: 00:25:32 Yeah, thank you. Uh, and thank you for the opportunity to chat on this topic. And I would just say eventually we're always happy to chat with customers or other folks who are interested in Benchling, so anybody can find out more at benchling.com/connect or I'm always happy to chat with folks. Our team are happy to chat with folks, so feel free to reach out with questions and or to chatting with you all and, uh, being at SLAS, next year.
Hannah Rosen: 00:25:54 Great.Thank you so much.
Glenwood Barbee: 00:25:55 Thank you.