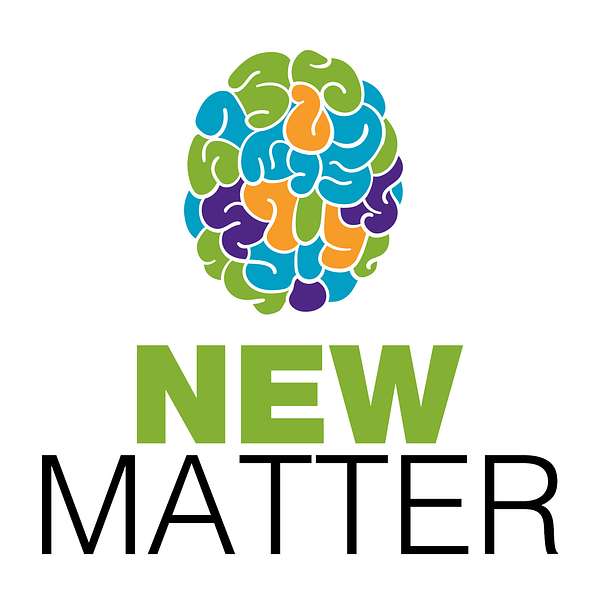
New Matter: Inside the Minds of SLAS Scientists
The official Society for Laboratory Automation and Screening (SLAS) podcast explores advances in automation, cellular imaging, big data and what's coming in the spaces between traditional scientific disciplines. Guests often include members of SLAS along with innovators, leading experts and other members of the global scientific community to highlight technology and even career stories. Episodes are released every week and subscribe to New Matter - available on all podcast players.
New Matter: Inside the Minds of SLAS Scientists
Lab of the Future | Data Management with Oliver Leven, Ph.D. and Sascha Fischer, M.Sc.
In this installment of our "Lab of the Future" series, we take a look at how data management is evolving as laboratory technology continues to advance. Our guest is Genedata Head of Strategic Operations Oliver Leven, Ph.D., and Business Development Manager – Automation Sascha Fischer, M.Sc., to share their perspectives on data management and what Genedata is contributing to the Lab of the Future.
Key Learning Points:
- Changes in data management technologies
- How "FAIR" applies to data management
- Important configurations in data processing workflows with AI
- The role of automation in increasing data quality
Full transcript available on Buzzsprout.
Take our Podcast Survey!
We want to hear from YOU to ensure we provide valuable content that reflects what our listeners and the SLAS community are interested in! Take our brief survey to help us learn how we can improve New Matter.
https://www.surveymonkey.com/r/BD7BC82
Stay connected with SLAS
About SLAS
SLAS (Society for Laboratory Automation and Screening) is an international professional society of academic, industry and government life sciences researchers and the developers and providers of laboratory automation technology. The SLAS mission is to bring together researchers in academia, industry and government to advance life sciences discovery and technology via education, knowledge exchange and global community building.
SLAS2026 International Conference & Exhibition
- February 7-11, 2026
- Boston, MA
SLAS Europe 2026 Conference and Exhibition
- 19-21 May 2026
- Vienna, Austria
Hannah Rosen:
Hello everyone and welcome to new matter, the SLAS podcast where we interview life science luminaries. I'm your host Hannah Rosen, and today we'll be continuing our series focusing on the lab of the future with Oliver Levin and Sasha Fisher of Genedata. Genedata was one of our SLAS2023 Lab of the Future companies and they're joining us today to discuss managing data in the Lab of the Future. Welcome to the podcast, Oliver and Sasha.
Sascha Fischer:
Thank you very much for having us.
Oliver Leven:
Thank you.
Hannah Rosen:
It's our pleasure. So to start us off, I would love it if each of you could maybe just kind of give us a little bit of your professional background and what it is you do at Genedata. So, Oliver, would you like to start?
Oliver Leven:
Yeah, sure. I studied chemistry, and after that I achieved a PhD in bioinformatics. I should say ohh, that is more than 20 years ago, so quite a while now. I've since 20 years at Genedata. And I always have been working or most of the time I've been working with the Genedata Screener product, and in this product I've been focusing on the automation of data analysis. So, by connect to the data analysis existing automation platforms like, for instance, what you are doing high throughput screening. So focus on automation has always been the focus around, yeah.
Sascha Fischer:
I studied molecular medicine and started my career as an applications specialist mainly focusing on NGS and genomics applications working for Hamilton and Tecan, and I joined Genedata around, a little bit longer than two years ago and now I'm in a business development role and here my focus is on and working with customers and partners and on developing solutions to automate highly complex experimental workflows where mainly Genedata's role is in experiment data capture, data processing, integration, as well as data analytics and reporting.
Hannah Rosen:
So can you tell us a little bit about, you know, a.ittle bit more detail about what it is that Genedata does and how is Genedata as a whole viewing this concept of the Lab of the Future?
Oliver Leven:
Yeah, sure. The lab of the future. Of course, the first question that comes to mind, I mentioned I'm a long time in the area, in the field, and the first thing that comes to mind is what is the future? What you what is the time frame we are talking about? So let's assume we talk about 10 years, and let's talk about what will change in the lab part. So what will it make different from what it is today? And then there's one thing which stands out will be much more automated, right. Already today some experiments or some workflows are being automated and we've seen increasing pickups, so a lot of things are being automated, which means individual experiments are automated. The question what is currently being automated is, it's mainly dependent on the economic factors. For instance, how frequent, how standardize, or how complex are the experiments which are being run out. And we know that this is going to happen and we see the pickup first hand, right, because many of our customers have their automation platforms integrated with our software platform, what we call the Genedata Biopharma Platform. And now coming back in 10 years, we expect, I would say all but close to all such components to be automated, right? And the manual running of experiment is the exception, of course. The last phase is always the last 20% of the experiments to be automated. They are the most complex one. That's also they take the biggest investment, so maybe take a little longer than the 10 years, but overall we think in the level of the future, scientists wouldn't touch things so much anymore is what we all be automated.
Sascha Fischer:
So what we do see is actually that in biopharma R&D manufacturing there has been made a tremendous investment, large investment in automating workflows, right. And I think the idea here was to be less dependent on human resources, right? Scaling was for sure, throughput needs, scaling was a very important aspect, and also, you know, the reduction of the human error and biases. And I think that all results and then saving and time and costs right, I think that was the ultimate aim. You're right, but I think what has been a little bit neglected is that huge amounts of complex raw data is already generated today, and even more in future, right. And we do see that if the automated platforms are not enabled to handle this large amount of data, you create a lot of added value automating that lab processes, right, and didn't limit it because the automation data handling is not there. What you're talking about here is for sure large amount, complex data which needs to be processed, analyzed and actually expected there should be little or no human intervention be part of that. And, you know, subsequently result into an efficient decision making process, right? Genedata is a business that is built in providing, what we like to say in digital backbone for biopharma organizations, right. And our aim is to automate the capturing processing and analyze the terabytes of data, of complex data, without human intervention. And here we do follow a concept which we named an exception handling, right. And what does that concept means is there is an automation and data handling unless there is the situation that an unexpected result is generated, right? This is actually the one result which requires a scientist intervention, right? And this is a strategy or a concept you're following, and this requires an extremely deep understanding of, you know, the technology, the science, the experimental workflows. And this is why we at the moment are focused in particular on the tight integration with analysis technologies, taction instruments and the robotic systems.
Oliver Leven:
So what we are doing that in data is what we call we provide the digital backbone to our customers, right? So that means we provide an open, scalable, integrated infrastructure for their biological workflows. By doing so, we believe or our customers actually prove that experimental workflows and the execution there can be speed up tremendously, right, by orders of magnitude. Because if well set up it eliminates unneeded room intervention, right? Think about that. Somebody has to manipulate a file or take a set of files and throw it into a folder or do some edits in Excel or something like that, right? Or hand over thresholding quality assessments that somebody has to look at some outcome and say yeah, well that's good or maybe it's not so good. And for that, what we see is sometimes our customers have spent days for the data analysis, right, and that can be done in hours or even in minutes. And so sometimes you also see that projects, they simply store because the next step is to be done by a colleague and then is sitting in the colleagues inbox, right. And so he doesn't process because for some reason he has read the inbox, the e-mail, but not reacted to it. So what we see is that for this digital backbone as we call it, we wanna eliminate human intervention if it's not needed, right. So because that opens the door to scalability and it allows us to run more experiments in parallel or another little detail or an important aspect of this is that of course, by having an awfully automated data analysis that also increases the data quality. And it does so automatically because it eliminates the human error in the bios, and that is a big value on its own.
Hannah Rosen:
I'm curious, you know, you've talked a little bit about, and this is a common theme that we hear, is as labs become more automated, the amounts of data that are being produced just seems like it's increasing exponentially. And so you've kind of alluded to this a little bit, but can you go into a little more detail of, you know, how are data management needs changing as we look to this Lab of the Future, as things are becoming more automated and, you know, what are some of the things that maybe people aren't thinking about or aren't aware of as our data management is shifting from a more traditional view of how to analyze and manage data?
Sascha Fischer:
Yeah, yeah, I think what really needed us, really quiet is bringing together and the broad range of different technologies, right, and enable that they all digitally connected and integrated with each other, right? We do see because this is actually the title of the podcast that the foundation for such a lab of the future will be structured data management. As already said, you know, with data captured from the technology properly and put in the right context, is integrated. Appropriate processing steps will follow and an analysis with either sophisticated algorithms. And finally, also that the results then will be automatically reported and this should ultimately result into a decision which are proposed as an ultimate goal, should lead to a faster and better decision making, enablement of that, right.
Oliver Leven:
If you look at data management for a long time, there's a term FAIR came up, right, data should be findable, it should be accessible, interoperable and reusable. Basically that data should not get dusty in the shelves of some database, right? It should be accessible whenever it's needed. That's basically the background behind FAIR and that's good. That's all FAIR. However, what Genedata now is looking at is what we call beyond FAIR, right, and this is, we have to look beyond the individual experiment beyond the individual workflow, right? So we need to integrate the longer portion, ideally the whole of the workflow and that requires that we have proper data models, right. But also we have to make sure that the data which sits in the data models, the raw data and the results, right, that they are produced and checked by sound state-of-the-art algorithms, right? And that we're not just focusing always on the individual experiment, right. And this is one of the big strengths which our software provides because it provides us a little background, the backbone, the whole digital backbone, and that is something which you cannot achieve if you're just running point solution, interview solutions or maybe even just electronic lab notebook.
Hannah Rosen:
Yeah, it's interesting that you bring up the whole FAIR concept. I'm curious, and this may be jumping ahead in the discussion a little bit, but it just brings to mind, you know, a lot of times when we're talking about data management of these automated processes and the large quantities of data, and especially once we start integrating AI into this data management, we talk more and more about how we are not in the end going to have access to or see all of the data that is produced simply because it's too much for humans to deal with. How does that, do you think, play into this FAIR concept of making the data findable and accessible, if even the people who are running the experiments may not be accessing all of the data?
Oliver Leven:
First of all, I would say it's not the data volume which makes things complex, right, it's the question of how transparent is the raw data which has been recorded, what is the meaning of that data and how easy it is for you as a scientist to access that, right. We support many different technologies and some of them, they produce gigabytes or terabytes of data. And nobody would be looking at this log data because you can't see it. At least you would not be looking at the raw data without an appropriate viewer, which gives you the accurate representation of this data, so that you will have all the data. But you see just an high level view where you can make out the structure, where you can make out the connections, where you can do some of all the necessary tracks which you have to do, but then also which allows you to go down to the individual measurements to really resolve and zoom in. And this is what then the tool chain or what the software is. Our software, what it provides, to give you both the overview as well as the possibility to zoom in and to eliminate or identify some tiny issue which might be somewhere in your raw data causing the aggregated data to not meet some quality standards. So it's not so much about the data volume, it's also about the complexity of the data which you're looking at, right. We can easily imagine that you have very simple lean data, but it's really complex because there's so many dimensions. There's so many dependencies and just looking at the scientists at this table, you think about those numbers, a lot of numbers in order to make sense of this, you need always visualizations you need. Algorithms which aggregates the data, allowing you to see the view which you need to understand the experiment.
Sascha Fischer:
And I think what you also can add here is, you know, that the input data provided to create AI models and following the FAIR principles, meaning, you know, what Oliver mentioned at the beginning, the sheer amount of data will increase and probably not everybody will at look at every raw data point anymore, right? But what we are convinced that accessibility to use raw data points should be enabled and guaranteed and made available, right? This means if you have for sure a large data set which you train your algorithm or the AI algorithm is being trained, right, you know that binds a little bit in what we discussed a minute before unexpected reviewing concept that we were talking about, meaning as soon as the data become not clear anymore or the output data or the result data become not clear anymore and there should be the availability or the accessibility or the findability of the raw data to actually check for the root cause of the problem. More often, you know, unexpected results, and I think is the real expected by the scientist community to have an enablement in that direction.
Hannah Rosen:
Yeah, absolutely. I'm sure that'll be reassuring for some people, cause I think a lot of times when you hear about the way that AI is going to be processing our data, it brings to mind this idea of just the AI is going to tell you, OK here's what you should do next, and you just gotta blindly trust the AI. But it's nice that it is not going to be such a black box that you can't dig in and figure out how the AI came to those conclusions.
Sascha Fischer:
That would be, for sure, yeah, absolutely the way to go to give the solutions the trust needed to be applied also you know in critical environments, R&D etcetera is definitely, yeah.
Oliver Leven:
I think, independent of the methods that you use to analyze your data, you as a scientist, you're in charge, right? If you say if I just use the algorithm, this is the result, then of course you might feel some quality you might have applied the wrong algorithm, right? So that's pretty common in curve fitting, right? You fit five different models to your curve and then you pick the model which fits the data. But what you should do as a scientist, you should go in thinking of an understanding on what is the expected biologic behavior, and then you should take the model which matches this behavior and then it should say if your data describes this model or if it doesn't, and maybe if it's statistically not valid, then maybe your process is wrong. Then you can maybe try another model or maybe you have to repeat the experiment because there was maybe too much noise, too much error on top of it, but in the end of the day, as a scientist who are in charge of this whole process, you cannot lean back and simply say oh yeah, these are the results, I don't know how they came up or not. You have the responsibility. You have to sign your results in the lab notebook. You have to report to your boss, possinily millions are depending on on your outcome. So basically means you with your credibility as a scientist, you have to understand what this is about and you have to explain this.
Hannah Rosen:
Yeah, it reminds me of someone that we spoke to recently on the podcast on a similar topic who's describing AI as sort of like a a low level lab tech or like an undergrad or even a high school intern where it's like, they can do some things, but you probably wanna be checking their work just to make sure.
Sascha Fischer:
Yeah. Definitely. Absolutely. Absolutely. Yeah.
Hannah Rosen:
Can you guys go into a little bit more detail of, you know, we've been throwing around the AI a lot so far in this conversation? What role does and will AI play in data management as we move further and further into automation becoming more common and will it eventually be possible to have data workflow management without having AI involved?
Sascha Fischer:
Yeah, I mean, we at Genedata, we do see that the arrival of artificial intelligence is extremely exciting for sure, right, also for us, right. And actually the Genedata biopharma tool, that one which we have introduced a minute ago is seen as a foundation to enable our customers to apply AI approaches in the future, right? What actually we want to achieve with the platform is that it allows our customers to, as we said, you know, generate the structured high quality data sets which are needed for the deep learning AI based approaches. And these high quality learnings are required for successful AI. Yeah, this is something we see that there is a demand to be generated in the industry as the first steps, right. We do see also that, uh, currently, the lack of such high quality data for providing sufficient learning data sets are holding up super often the successful implementation of artificial intelligence, right. The second approach is that we want to enable our customers to bring further their own AI approaches and, you know, asset, you know, help them to automate the data workflows and we do also have several projects at the moment underway where we are aiming to provide interfaces for easier integration of AI tools. We are can provide more news around that in future, not today. Finally, we do see a lot of quick ones which can already be gained by implementing AI, right. It can assist daily operations in the lab. It can help you to identify process flows, procedure, but it also is sure implemented and a good approach for long term improvement and generating novel insights, right. And here we do see examples, for instance, such as develop ability of anti antibody candidate or detect new phenotypes when applying it to microscopic, images. So yeah, that's actually how we can see that this comes to.
Oliver Leven:
I just would like to come back to your second question. So basically, which is if I got you right, will it be possible to have data workloads, workflow management without AI? And I think here clearly our answer is of course, definitely, right. If you think about the data workflow, what does it mean, right? It means you have data which is stored and you know what data is stored. Maybe raw data can be a result of one experiment can be different. Bigger entities, right? And then to come from one entity to another entity. What you have to do some sort of transition, some type of analysis, some sort of aggregation, some sort of decision making, right. And some of these steps will be driven or will be improved by using AI, right? But that means the workflow itself doesn't need AI, right? And where you have a tool which is good enough, you also don't have to throw AI at it unless it's much better, right? So actually, what Genedata has in more than 25 years is we have building data management and analysis platform, right? Long before AI or, as some say yeah, machine learning, we've been doing machine learning in the 80s, right? And all this is based, as far as Genedata and AI is not just something new and I don't want to don't play the role of AI, but certainly it's a tool, and can be applied in certain steps and sometimes makes sense, and sometimes maybe it doesn't, right? So that means what we believe in is that you have to model the biological processes, right. And you have to use strong statistics, a good foundation, you know, to make sure that your results are actually the results you're looking for. And such platform data has built, will continue to improve in the future as there's no doubt about that. On the other hand, AI is there, it's picking up, it's becoming more and more usable and more and more easy to be used to be applied, right? And whenever there is a possibility to deliver our advantage to our customers and to give you one example, we have a product called Imagence, and this is actually AI, so ar convoluted neural networks to identify biological phenotypes in high content screening, which is a tricky task and Imagence makes it really simple. And so this, for instance, example where we use AI and there are many more.
Hannah Rosen:
Sasha, you had mentioned that a lot of the times the issue is with the quality of the the data training sets for AI. Could you talk a little bit more of, you know, what is commonly the issue there and what can we do to improve the quality of these training data sets?
Sascha Fischer:
Many aspects, right? I think what actually labs should be developing is in solid data management strategy, right? And what we see very, very often is that there is a lot of initiatives on going to set up a data management strategy also on an enterprise or organizational level. But, you know, what we always learn is that it is the change management process, right, and how actually scientists think about and record their data properly, right? It is often not thought about the value which specific data can bring for other parts of the organization, right, or what it can be in the long run that this data can be of high value, right? And this is often a little bit neglected or not recognized by the individual. So that means times is often focused on getting to the next steps and their mental workflow, right, thinking about more in that direction instead of what is the need of our reorganization over a longer period of time. And therefore we think here it's really like change management or change of thinking process needed, right. One solution is for sure that all that data needs to be captured in digital system which, you know, can serve then not only as a foundation for the AI but also to improve the quality of the data and, yeah, our biopharma platform is an actually very good start to enable such a solid data management strategy. And what we are also doing very often is we are partnering and support our customers to set up such a foundation for their labs for the processes to help them to, you know, put such a data management strategy in place and also then organically help them to transition into AI application. And I think here is really like the key for the power dictation and thinking data and recording data.
Hannah Rosen:
So it sounds like it's a big part of this is preparing for transitioning into this method of data management before you actually do it. So there's labs out there who maybe aren't currently ready to transition into using AI, but they should be taking some of these steps in order to make sure they're ready for when that day comes.
Sascha Fischer:
Absolutely, yeah. Because I think what is more and more, the awareness that the gold market, so to say are in the data, right, and everybody is talking about and I don't dare to say it loud, the big data and what to do with it, right? So I know it's a little bit of buzz words here, but actually the idea, you know, to happen long term strategy with big data, making them drawn for being good, high quality training data sets for their application. There is no way around because I think when talking to data scientists there is a large amount in the day to, you know, making sense out of the water that they have and, you know, kind of putting some magic on it to kind of clean it and sort it and make it adaptable. And yeah, this for sure can be changed if everybody in the organization takes responsibility to follow such a strategy, a long term strategy. And I think this is actually a very good approach and the key of the success for future AI implementation and all.
Oliver Leven:
I would also like to point out how AI can deliver or actually improve data quality, right? Because there are certainly ways and possibilities to do so. So for instance, if you think about biological research there often it's experiments are done, assays are being performed, and basically what you do is, you will describe a ideal behavior of your biological samples, right? So and then you test right and at end of a test you get a result, right? And then you typically use a thresholding approach. That means you take the 10 best percent, whatever best means, right. If you know things that you have a simple outcome, right, a single number, then of course the thresholding is simply straightforward. And that's one of the case, for instance, where you say you would not need AI or something, but think about it much more complex thing about not just a single number, but there are 5000, 500 numbers for each of your samples. So think about the whole image, like before, the millions of pixels described the result how do you do this then, right? Because it's not a single number you can use for threshold, right? And the reason why we see AI being picking up here is that some of the older applications that are used it only works to limited extent. So for complexity with this, they're being used for instance, like principal component analysis and other statistical approaches which just sound which are fine, they're working really well. But they don't work for that over complex data. So what you do then instead of this is you turn it around, right. First of all, you run control samples. Basically you manipulate your samples in the way that you can that you can control, that you understand. And then you'll get the outcomes of these control samples and then use these outcomes to train the network and then this network then can classify your samples or can identify bad samples, bad measurements and so forth, right, and that works pretty well. And this is what Genedata is developing together with customer, but it is was a very isolated focus on a very specific type of experiment.
Hannah Rosen:
So it sounds like there's kind of two approaches, there's the approach of the kind of broader overall lab process, look at the data management, and then there's the individual assay or experiment’s data management.
Oliver Leven:
And of course, they have to be hand in hand, right. You see a lot of people right now working on the individual ones, but and then they got nice individual solutions for some point problems, but they have not started to pick up, which is what Sascha was talking about.
Hannah Rosen:
Interesting so, you know, we've kind of talked a little bit about all of the different ways that AI is being integrated and will continue to be integrated into data management and workflows. Can you go into a little bit about, you know, how will utilizing AI in data management improve these workflows, in data analysis specifically about, you know, using AI as a tool to analyze all that massive quantity and complex data that is being produced?
Sascha Fischer:
You know AI to improve data quality requires first of all proper IT backbone. It requires structured and high quality training data. And I think what is also important to mention here is that AI can never be seen as done, right. It's something which is steadily proceeding and in progress, right. So the models are trained, they need to be retrained, they need to be optimized again and whenever there is new experimental data generated, right? This also means that, you know, that there is a tremendous import to know which version of a specific model you have worked with and what was used to produce the result. So to say, also follow up versioning approach here that is actually coming back to the platform, the Genedata Biopharma Platform, what we can provide, you know, really like, to have enabled these high quality structured data which you can backtrack and follow up also over different versioning. This gives you so to say the security to be future proof and also, so to say, have the chance to check back what has been generated.
Oliver Leven:
Yeah, I think that also goes a little bit back to your earlier question. So how much of the actual raw data can the customer see, or the initial data, can the user see? And this we answered already, but then also as Sascha touched on is what is the AI solution which was applied, where did this come from, what is a data set the AI was trained on? Just think about, you know, ten data sets. And you have trained the model and now you're applying it. Of course you do also statistical test to make sure that the classification done by the area that there is statistically sound, right. But now let's assume you have no 11, 12 of the 13 data sets of the similar experiment, which you can use now to retrain the model. So, if the outcome is still the same, then likely you don't bother, but what you do is the outcome is. So there's always this, you have to see the raw data you have to see the model. You have to understand how the model has been trained in order to really understand the outcome of it. And of course, always you have this authority of the scientists to say that it was not just blind guess right, but it was a prediction which is statistically sound.
Hannah Rosen:
What about the limitations of using AI in this way? You know, clearly you're saying you were still going to need the scientists to go in and check the data so, you know, what are some of the, maybe the downsides or just the limitations of using AI and how will we need scientists to continue to step-in in this process?
Sascha Fischer:
Yeah, I think, yeah, I see, I think the limitations is definitely, it can be seen as reducing workload for humans, right, and generating answers for standard questions, but they are extremely, often decisions need to be taken the lab where AI approaches are not of help, right. And we do see that the human judgment and the ability to think outside the box have unique benefits which cannot be achieved by AI. And therefore we are following the approach that AI needs to be seen as a tool and it helps to reduce redundant and repetitive tasks, right? But it will not be replaceable by humans. And yeah, we do always say the human creativity, there's nothing which can be replaced by AI and therefore we do see limits there.
Oliver Leven:
The request is always what is the util of this individual little step or the bigger step which is done by AI and how reliable is this step? I'm sure if I can bring an old story, but I was there then digital photography came up. I recall in my first pictures, which I had on my PC, that you had to select the eyes of the people and after you selected the eyes then it was automatically taking out the red, the red eyes, and then color them somehow, I don't know. That was something stupid which now I don't have to do anymore and I'm pretty grateful of that, that I don't have to do it with every photo. It's just done, right, and it's good, it looks well. And there are a task like this in the lab and tasks like this in data analysis and data classification which will be, they’re just nuisance, they are actually stress work. And this will go away, and this is a good thing. That, on the other hand, of course, now making the big jump and letting Siri propose my next meal to me based on what my refrigerator, I'm not sure of that she has it all together to really hit what I need. And so basically if this question becomes too big, if it's too bold to step into the future, then of course the scientist has to be wary and has to make sure that he really understands what's going on, right? Going back to this example, right, if I now look back at the last 10 years of photos, I don't care who and what colors the images, they're just correct, right. And similarly, that will play out with AI. So there will be applications that's just applied everywhere and nobody notice anymore. And there were others, which always there may be bigger prediction, bigger classifications, which maybe sometimes fail. And like you say, oh, no, we have to be really careful. And then you paddle back a little bit and then it goes, moves on again.
Hannah Rosen:
You had mentioned, kind of at the top of the podcast, that one of the big advantages of using AI or machine learning in these data management processes is going to make the data more reliable and more trustworthy and more consistent. I wonder, so one of the things that I hear a lot about the concerns with AI is that it has the potential to propagate human biases because we are creating the training sets essentially that it's going into there. And so I wonder if you could speak a little bit about what is your perspective on that potential of having human bias continued through machine learning, and what can we do to kind of prevent that?
Oliver Leven:
Yeah, in theory, the scientists should set up the experiment in a way that there's no bias, right? And that is what scientists all over the world are doing, right. You have to plan your experiment from the beginning, and bias is something which you learn in the second semester or something and you have to be really careful about this, right. So that are not just proving what you did anyway, so what you therefore have to do is, first you have to think about what is a biological question you answered. Then next question is then, OK, to answer this question, this biological question. So how would the experiment look like, which I have to decide. And that's all talking biology, that's talking about laboratory automation, that is chemistry, biochemistry or what you need to know that the cells behave and live and grow and be, prosper and so forth for it. Just for example, right, if you have yourself in an unhealthy condition and they're dying, that might not be due to the toxin you were developing, right, it might just be they were dying anyway, no matter what you did, so therefore you have to set up your experiment in the way that you have proper controls, which I mentioned before. So you have to, each condition you want to, if you have to first of all be able to produce this condition in a reproducible way, right? And if you do all of this, then, the bias of your experiment or of the AI which you train on this experiment is within your sample setup within the setup of your controls. So basically if you screwed up your control, and the drugs screwed up. Or if you learn later, more or something which you didn't understand before. And then the new fact, which are simply not known at the time point when you planned this experiment. But the science right, you try to come up with some sort of model describing the reality and then you did do an experiment and the proof, and in this step in this cycle, AI can be a bit of a shortcut because it takes away tedious manual data processing now, but at the end of the day it's the scientist to set this up, so therefore I don't see this type of bias happening. Of course, if you don't know any of this background, and if you're just without experience in the field, just take some model and apply it blindly well, things come out, but let's say this bias or the problems which are then occurred with something like this, they would also have occurred without AI, right? So it's just the tool used wrongly.
Sascha Fischer:
I cannot add that much to that. I mean, when you're, I know about what you're referring to, but I think we need to make it discrimination here between, you know, general AI tools which are available and interesting to the society, you know, image generating AIs, text generating a AIs, binding in political or religious statements that create a bias, and there is insurance, and I think there is a lot of examples which show the downside of a better training data and what the result can be right? But I'm following all of our statements here that actually scientists are dealing with scientific data, I think actually every scientist in his education on in the first year of university will have, so to say, the awareness created when you're designing a study, when you're creating data, when you're following an unproven approach and make sure it's best case not bias. We're sure we are not living in a unicorn, isolated, perfect world. Therefore there is definitely problems to solve as well. But in the end, I don't think that AI applications for scientists implemented could a little bit more overcome that topic. Compared to the general AI applications where we do see a lot of problems with that bias.
Hannah Rosen:
Yeah, I think that that makes sense. You know, it sounds a lot like, you know, as long as scientists are following the scientific method and not getting lazy and relying too much on the AI, they should be fine.
Oliver Leven:
Yes, yes, yes, definitely.
Sascha Fischer:
And again also, you know, on the accessibility of the raw data or also having critical, I mean, in the end, I think a good thumb up could also be AI tools, they need to be critical, right? I mean, there are so many unsolved questions and so many regulations need to brought in place, right? This is not only biased and also, you know, has the ownership of the data, with the ownership of the generated data based on the AI tools, we call it the train data, comes from another, there are millions of topics which are not, so we need to see this critical. Everything, you know, making sure to overcome the risk of bias is just one of these million topics. But yeah, they're plenty more and more, so careful usage of AI, see how it can be enabled in the laboratory to make one’s life easier to overcome redundancy, decrease workload, focus on the scientific question to have more time to read an interesting paper, etcetera. And yeah, I think this is how we should see it at the moment, right, to how people should apply it carefully.
Oliver Leven:
Of course you can foresee in real life is that, you know, you’re talking mainly research here, right? Which is, as we tend to say, far away from the patient, right, far away. But of course, the closer you could get with such experiments to the patient, right, you cannot simply then tell if they, yeah, this AI has said that this is a good drug, right. Or that this drug doesn't have any side effects or something like that, right. So there has to be a proper sound validation and this AI goes more and more also it will go towards this direction. We will, of course, need more regulation, but I'm pretty sure that the FDA will come up with regulations, will ensure that is well understood where and how AI can be applied, right.
Sascha Fischer:
And probably testing the outcome and the results of the solutions as well, right. And I think you're generated answer by an AI tool needs to be followed up in a proper testing and I think, yeah, this should be applied to guarantee the outcomes here.
Hannah Rosen:
Well, Sasha and Oliver, thank you both so much for joining me today. It's been a very fascinating conversation talking about, you know, how AI is changing our data management workflows for the lab of the future. And we just really look forward to seeing Genedata at more of our SLAS events and seeing what new solutions you guys come up with.
Sascha Fischer:
Thank you so much for having us, it was a pleasure to be here. Thank you.
Oliver Leven:
Thank you. And our pleasure and our side. Thak ou so much.