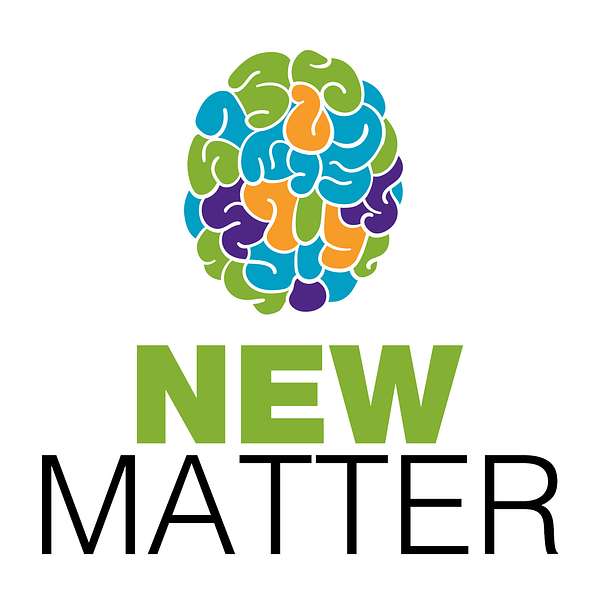
New Matter: Inside the Minds of SLAS Scientists
The official Society for Laboratory Automation and Screening (SLAS) podcast explores advances in automation, cellular imaging, big data and what's coming in the spaces between traditional scientific disciplines. Guests often include members of SLAS along with innovators, leading experts and other members of the global scientific community to highlight technology and even career stories. Episodes are released every week and subscribe to New Matter - available on all podcast players.
New Matter: Inside the Minds of SLAS Scientists
Lab of the Future | How Open-Source Accelerates Research with Jon Brennan-Badal
In this installment of our "Lab of the Future" series, we take a look at how open-source is changing the research landscape at a rapid pace. Our guest is Opentrons CEO Jon Brennan-Badal, who's here to share his perspectives on open-source technology in the life sciences and how Opentrons' latest product Opentrons Flex™ is designed to help researchers break new grounds in R&D.
Key Learning Points:
- The benefits of offering a product with open-source
- The future outlook of open-source-capable devices in biotech
- The advantages of using AI to generate protocols
- Trends in open-source technology within the life sciences industry
Full transcript available on Buzzsprout.
Take our Podcast Survey!
We want to hear from YOU to ensure we keep providing valuable content that reflects what our listeners and the SLAS community are interested in! Take our brief survey to help us learn how we can improve New Matter.
https://www.surveymonkey.com/r/BD7BC82
Stay connected with SLAS
About SLAS
SLAS (Society for Laboratory Automation and Screening) is an international professional society of academic, industry and government life sciences researchers and the developers and providers of laboratory automation technology. The SLAS mission is to bring together researchers in academia, industry and government to advance life sciences discovery and technology via education, knowledge exchange and global community building.
Upcoming SLAS Events:
SLAS Europe 2025 Conference and Exhibition
- 20-22 May 2025
- Hamburg, Germany
Hannah Rosen:
Hello everyone and welcome to new matter, the SLAS podcast where we interview life science luminaries. I'm your host Hannah Rosen, and today we'll be continuing our series focusing on The Lab of the Future with John Brennan Badal of Opentrons. John's joining us today to discuss Opentrons’ use of an open source and accessible API in their new Flex Robotics System. Welcome to the podcast, John.
Jon Brennan-Badal:
Thank you. Happy to be here.
Hannah Rosen:
And we're happy to have you. So to start us off, I'd love it if you could just kind of give us a little bit of your professional background and your areas of expertise.
Jon Brennan-Badal:
So my background really is is as a serial entrepreneur, previously I built up a company called Comixology, which is actually in a very different field than life science or robotics. It was actually a digital comic book company that I sold to Amazon almost a decade ago, but what that enabled was a I think, a really unique experience on building and scaling a. Start up. Repeating against and winning against companies like Amazon and Apple, and learning how to transform an ecosystem in that case, digital media and how comic books were read.
Hannah Rosen:
That's so interesting. So then what got you interested in the fields of lab automation?
Jon Brennan-Badal:
Yeah. And so I was continuing to operate that business as a part of Amazon. I wanted to go out and build another business, but try my hand at a larger, more complex set of problems which really led me to the life science space and in particular to robotics and liquid handling automation.
Hannah Rosen:
Wow, that's fascinating. So I mean, you know, when you got started, did you just try to dive in and try to learn as much as you could or were you just seeking out other people who had experience in the field and kind of relying on their areas of expertise to start off?
Jon Brennan-Badal:
Yeah, it really was a a combination of both, and so believe or not Opentrons actually started as a community project in a biohacker space in Brooklyn, where a group of individuals, both a combination of life scientists, mechanical engineers, software engineers from outside the life science industry and myself included, got together and built the first prototype Opentrons robot. And from kind of the get go, we really tried to incorporate kind of the right elements of best practices from life science with some of the more innovative approaches that we've seen kind of done kind of, you know more broadly across, you know, tech and startups.
Hannah Rosen:
So kind of trying to come at these problems of life sciences from almost an outsiders perspective?
Jon Brennan-Badal:
Absolutely.
Hannah Rosen:
So when you guys got started, did you have this idea of wanting to kind of shake up the life science industry a little bit and kind of push it into the next stage of laboratory automation? Or was it just sort of like a fun project? Did you, what were your goals when you started out?
Jon Brennan-Badal:
Yeah, no. I mean when it started initially as a community project, it really came out of the desire to automate some of the activities that we're performing in the lab. And given that this was a hobby at that point in time, we didn't want to spend a couple of $100,000 or more to kind of automate those activities. And so it did come out of that very kind of specific need. But through that kind of initial journey of building that prototype and evaluating the industry kind of as a whole, it became clear that there was a opportunity to build a much, you know, an actual business and something that could be, you know, highly impactful. And that's what led us to convert it from being a community project to a venture backed startup that could much more aggressively kind of attack some of the problems that we saw.
Hannah Rosen:
Wow, that's so interesting. It's such a unique and different entry point into this industry from what we typically hear and who we typically talk to. So this is going to be a really interesting conversation, I think. But that's so cool. So, you know, in the life science industry, especially in the automation field, we love to use this term nowadays of the lab of the future, it is one of the, you know, the hot topics that's being kicked around. And so I'm curious, you know, does Opentrons have a perspective of this concept of the lab of the future? And what is your approach in trying to, you know, develop products for this future lab?
Jon Brennan-Badal:
Absolutely. We definitely have a perspective of what the lab, or really labs of the future look like. And to kind of draw an analogy with, if you roll kind of back the clock on computers, let's say 30 or 40 years, and you're asking that same question, what is the computer of the future which might be like, you know, the computers of today? If you said any kind of specific example you'd be wrong. Because, well, computers aren't just the things that are on their desk. They're not the mainframes, you know, or that operate now kind of in the cloud. You know, they're not just the, you know, smartphone devices that are in your pocket. It's really the combination of all of those sets of form factors and more that represent kind of computers. And so as we kind of think about, what does the lab of the future, we really think about that as the labs of the future. Which is to say that we think that there's room and opportunity for, you know, Cloud Labs in which you can be really offloading all of that, you know execution to the cloud in a much more cost efficient, scalable and seamless way than say working with a traditional CRO.
But in the same kind of fashion, we also see very expansive, you know, highly automated on-prem lab operations that are greatly kind of scale in the ways that you kind of think about the natural kind of playing at the hand of biomanufacturing and bio foundries. But in kind of the same kind of vein, we also see different types of live experiences that are best suited to greatly enable rapid R&D work in small lab operations. And so in the same way that you find commonalities between all of the different form factors of computing devices, we think they're also going to be kind of commonality across. Those range of different kind of labs of the future and then we think that one commonality around that is going to be ubiquitous automation that's highly affordable. The easy and seamless reproducibility of lab work, and really the combination of both of those things, will also enable much more seamless transition between those different types of labs, because any type of project goes through a broader kind of, you know, life cycle first starting out in that smaller R&D lab or R&D labs, and may get scaled up to a larger kind of production setting, and ultimately may, you know, be outsourced to CRO's and then ultimately to some, you know, large, you know, bio manufacturing, you know, specialized kind of operation we see ourselves as providing some of that core infrastructure both in terms of that ubiquitous automation, but also increasingly enabling that easier reproduction. Our reproducibility of lab work across those kind of broader kind of journey and life cycles of any particular life science project.
Hannah Rosen:
Yeah. Ohh fantastic. So, you know, speaking of the services that you guys are providing, can you describe for us a little bit what is this flex robotic system that you guys have?
Jon Brennan-Badal:
Absolutely. And so we launched our new Flex robot, which is, a as the name kind of implies, a highly flexible liquid handling system and what makes it great is that it builds upon our prior work with the OTT robot, which is to say it is a highly affordable open source robotics platform that enables you to automate a wide range of workflows and with the various hardware modules in the flex robot, things like a gripper, a thermocycler, a 96 channel pipettor and more, you can use the flex system to automate very complex workflows including being able to bang out entire plates of NGS library prep and we believe by bringing down the fundamental cost of these types of systems by 70, 80% or more compared to competitive offerings and enabling a very kind of high-quality flexible system that this lays a key kind of set of infrastructure for that lab of the future.
Hannah Rosen:
Yeah, that's great. I mean, how were you able to decrease the price of your system so dramatically compared to other liquid handling robots?
Jon Brennan-Badal:
Yeah, absolutely. And so at the core of it, there was a lot of reimagining of robotic systems. And what that specifically means is that we designed all, not just the overall robotics system as a whole, but also redesigned and developed and manufacture ourselves, all the key components or subassemblies that go into these robotic systems. And that's something that's very unique to us in that we don't rely upon third parties or some assembly providers, we took on all of that. And by doing that, that has allowed us to take a much more holistic approach to the robotic design and develop novel approaches across all these different kind of components, which kind of all, when all brought together can drive down the fundamental cost of these systems by, you know, five or tenfold relative to competitors. So the short and long of it was a lot of hard work, but I think the flex system really starts showing kind of that pay off where you can now simultaneously have the performance of $200,000 system that starts at a price point starting at under $20,000 without the kind of trade-offs and capabilities or quality.
Hannah Rosen:
Yeah, I'm really interested in the fact that your, you know, system, it's all, it has an open source and the accessible API. Can you talk a little bit about what made you decide to go open source?
Jon Brennan-Badal:
And so as part of our long term view of that lab of the future, we saw a critical importance of seamlessly enabling reproducibility in science, and if you can greater enable that you can greatly reduce the friction associated with getting things done, because like right now it's such a pain. You're like oh, I bought this reagent kit I need to run and test it to see if it actually works in my lab with my automation setup, so on and so forth. And if you can, over time, kind of reduce those kind of friction points, we believe we can better enable scientists and that open source API strategy is a key kind of step towards enabling that. By having that open source API, you want better enable and scientists to be developing their protocols, making it much more seamless and easy for them to share and reproduce those protocols, and ultimately better foster a ecosystem or community in which your end users are building and validating all these sets of applications until ultimately you reach some critical, you know, critical mass where you can be leveraging your customers to be. Validating almost every imaginable application in the lab. That's kind of the key steps that we're slowly building towards and that that open source API was a key kind of piece towards building towards that longer term vision and opportunity.
Hannah Rosen:
Yeah. So is it almost as though like, now, you're giving researchers the opportunity to not just collaborate, you know, with people in their lab or people that they may know at other institutions. But it's almost like now anybody can collaborate with anyone else who's working on a similar project, as long as they're using this flex.
Jon Brennan-Badal:
Yes, that's absolutely correct. And we'll see is that the story of life science is really the long tail of all these unique different kind of applications within the lab because there's all these different specialized areas of research that all have specialized sets of needs and it's impossible for any one company to be able to meaningfully address and serve kind of all of those range of needs. I'll give you kind of a a fun example. One of our customers is a lab at the Mayo Clinic, specialized in gene editing for zebrafish. And one of the bottlenecks in their workflow really kind of centered around the time you had to wait for the zebrafish to grow from an embryo to something big enough that you could clip their fin and sequence it. You had to wait that time so that you could, you know, validate whatever gene editing work that you did, you know, occurred kind of as planned. And because of that, you basically would do your gene editing work, wait a couple of weeks and then you could do your sequencing work. And what that lab developed was a hardware module that agitates off individual cells off of zebrafish embryos so that instead of having to wait two weeks, you only have to wait a day and you can do single cell sequencing off of those embryos, and that collapsed kind of the cycle time for the set of work and that's something that was a hardware module that they built and developed. Concreted open source is something that was purpose built for our open-source robotics platform.
And so I'd give that kind of example of a kind of micro agitator for zebrafish. You know, single cell sequencing as a type of hardware module that no business could ever economically justify developing, but something that a highly innovative research lab is developing as part of its critics, pushing the boundaries of what's possible and driving kind of efficiency of their lab operations. And that's exactly the kind of innovations that can be, you know, enabled in mass across all these different kind of educates across the life science industry and it's harnessing and empowering all of those scientists to develop new types of applications and solutions like this or enable them to be able to leverage those types of innovations across all the other labs. In the world. And that's kind of what we see is the crux of the value of that open source API.
Hannah Rosen:
Yeah, it's great. It's like it's really taking these technological innovations and putting it back in the researchers hands where they're not relying and waiting and hoping that some provider is going to come out with the tools and the technology that they need. That they can kind of work together and develop it on their own.
Jon Brennan-Badal:
Mm-hmm. Absolutely.
Hannah Rosen:
Are there any other major benefits that you've seen going with the open API?
Jon Brennan-Badal:
So you know, the second piece by having an open API means that you have many of your customers building, working, contributing and improving that API. And So what that means is that even if you're a, let's say, a large company, you know, in biopharma that may not be as kind of, you know, focused on contributing kind of open source. You benefit from having a much more robust API in which you can use our tools to integrate in with your internal, you know, platform and kind of solutions. The second thing I'd kind of highlight is is that by having an open API. You not only have a higher quality API. But you, can more easily take advantage of broader technology trends that are occurring around the world, and I'll give you kind of the example of LIMs or large language models of which open API's ChatGPT or GPT 4 is kind of one of the kind of, you know, kind of high profile examples. Because we're the only robustly developed and open source API within the life science industry.
By extension, we're the only platform that has been training, ChatGPT or GPT 4 to be able to program and work seamlessly with our robotics platform. That type of experience is only made possible by having an open API. One, because you automatically are much more easily and so it's easier to be kind of have those algorithms, you know, trained on your system. Second, because you're open source, you have many of these different protocols or applications that have been developed which service as a corpus or training data to further kind of improve the performance of these types of systems. And so without having to make a very large kind of investment outlay. We're able to kind of benefit and easily incorporate some of these kind of really cutting edge technologies and actually have that work in extremely kind of high performance. And I suspect that as tech, broadly speaking, continues to kind of innovate over the coming decades, that there's going to be other technology trends that by virtue of having an open source API, you can uniquely benefit in a way that if you have a closed API or less robustly built out public facing API. That you won't be able to take advantage of, or only with great expense and time spent, which then, you know, leaves you kind of behind the curve.
Hannah Rosen:
Yeah, I'd love to hear a little bit more about what you're saying with being able to integrate the AI and these large language models into your API. You know, can you kind of go into a little more detail of what are the current capabilities of using those features, and how do you see it improving in the future?
Jon Brennan-Badal:
So first our API because it's open source and widely used, is extremely well documented, is based on Python. And because of those two sets of things combined with a very large community that's developed all these different, you know, applications for our system, all those are inputs into systems like GPT 4, and by training GPT 4 on that API, with that robust corpus, you can leverage GPT 4 to be very effective at programming the robot. Such that giving natural language prompts to compose a protocol can be done with extremely high accuracy, or which is even more kind of significant, I would argue is the ability to convert your manual process because every lab is doing activities manually. Manually to some you know some degree and by extension you probably have some written down document in a PDF or, you know, or what have you of what your protocol is or what your SOP is. Because GPT 4 is very good at converting natural language into Python and specifically our Python API because it's been so well trained on it, it can do a very accurate job of converting that manual, SOP into a machine readable file, and so these are kind of, you know, the examples of kind of unlocks that are made possible now to just greatly reduce the friction associated with converting your manual process to be an automated, you know, function in the lab. And you can kind of play that handout, as you reduce the friction to automation and while simultaneously reducing the fundamental cost of automation. That's kind of the 1-2 punch to enable automation to be more, far more ubiquitous across, you know, labs worldwide.
Hannah Rosen:
Yeah, I mean, I'm sure there are so many people out there, who love the idea of just being able to like, copy and paste their manual process in and have it spit out like, OK, here's where your automated process is. You're done. Don't worry about it. I mean, my goodness.
Jon Brennan-Badal:
And so as you know, and you know, because these technologies continue to improve because our ecosystem gets bigger and bigger, the accuracy of these types of activities continue to improve. You know, such to that, you know, right now you can have, I think a high degree of confidence, you know, in using that type of tool to program our robot for, you know, many common activities and in the lab and as time goes on, you know, there'll be broader and broader or more complex activities that you can kind of entrust it to do with an extremely high degree of accuracy.
Hannah Rosen:
Speaking of that, do you think, is it currently capable or do you think it will be soon capable of suggesting modifications to a protocol? If you run an experiment and pull that data and feed it back into say, like, GPT 4, will it then be able to say like Oh well, maybe you should try adjusting this or, you know, you could do this differently. Is it going to offer suggestions for the protocols?
Jon Brennan-Badal:
Because we have a robust, a very robust API. Our API actually already does that for common errors or common optimizations to one’s kind of protocol. And we've also trained GPT 4 to read those suggestions from our API and incorporate those into the protocols. And so I think as you play the hand out I think it's very kind of clear that much more complex sets of optimizations and suggestions can be made by technologies like GPT 4 or its successors. But what I would really kind of argue that the real kind of opportunity centers around GPT 4 used with our robotics platform with other pieces of software that might be used in design of experiments or other software built by life scientists that serve as a kind of a specialized agent for GPT. And so I'll give kind of the example, right.
So GPT 4, the technology is, I would kind of characterize it as a absurdly well read and articulate high school student that knows a bit about everything but doesn't have deep, deep kind of mastery. If you use GPT, you know, for all on its own, it's like, OK at math, but you're not going to be using it to do complex mathematical proofs. But when you connect GPT 4 with a complex agent like ,let's say Wolfram Alpha, which is a very kind of robust set of, you know, specialized software that's very kind of smart at doing, that's where it's able to leverage its reasoning ability and its usage of this type of tool to solve very complex mathematical problems, same sort of thing. If when you connect GPT or with our API functioning as that specialized age and now it gains that superpower of being able to effectively easily use our robot. In the same kind of fashion, many of our customers have developed specialized design of experiment software as part of just their, you know, pushing the bounds of what's you know possible, and that might exist in a, you know, a simpler form of like, Oh well, we take the output of this experiment and feed it in to modify it for our next experiment. And that's done through some, you know, in Excel or some of our customers have built complex AI and machine learning algorithms to do that type of type of work, but whatever kind of form that is, we've been finding is that customers have been connecting in that software with our software and GPT and other similar types of technologies. And you're enabling far more kind of robust type of applications.
I'll give you kind of this specific example of a lab out of Carnegie Mellon where they use GPT to connect and control our robot. But they also use that software to connect to their design and experiment software, such that you can now enter in a chemical compound that you want synthesized, and their design of experiment software will work through and make you know recommendations around, you know, what might be the appropriate. Kind of protocol that our robot can run. And then that can be kind of fed back to ultimately kind of search and kind of find the most kind of efficient, you know, synthesis path went. And these types of kind of closed loop solutions are easily kind of connected together, because GPT 4 is used as a connective glue between one interfacing with their design of experiment software. But having that design of experiment software interface with our robot and enable that kind of overall closed loop system where now robot because also their work at Carnegie Mellon was all concrete open source. You can now use our robot to, you know, synthesize a wide range of different kind of chemicals. And using that software you can figure out how to synthesize various chemicals through, you know, the processes that that system takes and so what I think will really see is how do we supercharge all the kind of experimental design software or other techniques that various labs have built and developed, and make it very easily to have that connected into integrated and highly automated systems. Because I can't tell you how many of our customers have, you know, in some degree or other some, you know, automated process to design their experiment or optimize their experiment and that software just spits out a way in which they can manually execute and this easily enabling that entire system to be highly automated. You can iterate that much faster and therefore find a much more kind of optimal solution to whatever is just like, you know, thing that you're studying or evaluating.
Hannah Rosen:
Yeah, I'm very, you know, you talk about with such a highly automated system and workflow. So where do you see the human element being integrated into this. You know, if we've got all of these different softwares and hardwares that are communicating with each other, and these closed loop systems. Where do you see the human researcher being integrated into these processes and, you know, how often do you get the pushback of people with that, you know, common concern of AI's gonna replace me in the lab?
Jon Brennan-Badal:
No, totally. And so, you know, we found because of our high degree of affordability, it's often been the lab technicians, the postdocs, not the lab managers or executives at at the organizations that are our biggest advocates is ultimately they want to enable themselves to be more productive and focus on kind of higher value activities, you know, as I kind of, you know, it points you with like, you know, GPT 4, it's like it's a very kind of a really remarkable kind of, you know, a set of technology. But it's like I said, it's like a really articulate and knowledgeable high school in terms of its complexity of reasoning, right. All these technologies enable is us to free up our time to work on the harder, more complex problems. When you kind of look at particularly the set of problems that we're looking at and leveraging of these technologies or reducing the friction to be able to get more lab work done with less, you know, resources being used.
And when we look and when we think about some of the large problems that like we as society or like life science in general wants to be kind of tackling over the next few decades, I would argue that it's much more likely that we need to be doing orders of magnitude more sequencing, more like orders of magnitude more, you know, production of synthetic DNA or all or various other kind of activities not like ohh over the next. Like can we do 20% more and if we could just do 20% more, we'll cure all of cancer, right? Right. The problem is we actually have are like, how do we generate, like orders of magnitude larger data set? And given that the number of scientists you know in the world is growing at like 3 or 4% a year and not like 100% every year, if you want to get, you know, orders of magnitude more data to be able to solve some of these hard problems, well, you need to be able to do orders of magnitude more lab work with essentially the same number of people, so fundamentally we need to be enabling automation to be fundamentally more affordable and ubiquitous and so and I think so many of our customers, you know those and scientists, those stocks, those lab technicians feel that need and see this as something that empowers them rather than, you know, replaces them.
Hannah Rosen:
Are you seeing any disadvantages or drawbacks to going with this open source API?
Jon Brennan-Badal:
It looks like, you know, everything, you know, there's everything is kind of a double edged sword, you know, and so, you know, look because you're open source, everybody can see what you're up to. And so that we were coming out with a new robot, really wasn’t a secret to any of our competitors now, I think if you're any reasonable company you probably assume that every company is making like the next new thing. So maybe it's not like, you know, so deeply, you know, like, surprising. But everybody including your competitors also get, there's much greater kind of level of transparency and so that is something that, you know, one should be kind of aware of. And so you want to be very kind of intentional about what you open source, how you open source it and if open source or just having a very well documented public API is the right kind of pathway given whatever your business strategy is. But given that we want it to be enabling scientists as much as possible and give them the most permission to do what they want, how they want it made sense for us to go down a very kind of highly open, you know, source approach with our company.
Hannah Rosen:
Yeah, I wonder with this, you know, all of the freedom that having an open source API gives you, does it also, how does that affect the learning curve essentially for wanting to use this product? Do you, is it a requirement essentially that your customers need to know a fair amount of coding going in in order to be able to fully take advantage of this flex robotic system?
Jon Brennan-Badal:
Ohh, not at all right? And so on top of that open source API we built a whole suite of software on top of that with great graphical interface interfaces so that you never even have to know what code is. You can just drag and drop and build your protocols that way. And in a similar fashion because you have a robust community that's built and validated so many different applications customers can use and build from that not have to worry about building it from scratch, which is kind of the kind of standard within kind of the industry. And so an open source API enables you to simultaneously better empower your technical users that have very kind of specialized or technical capabilities. But also it better enables you to serve the less technically savvy customers that are new to automation, have no knowledge, and have no desire to learn about programming right and that, you know, GPT, you know, 4 based experiences is a great kind of example of that where this highly technical API can be completely abstracted away through some of the most kind of natural type of, you know, interfaces of just write down a, you know, write down what you know any way you so choose what you want and it you know the correct file you know materializes.
Hannah Rosen:
How do you think, you know, you talked a little bit about the drawbacks of being that everybody can see what you're doing at all times and so, you know, for that reason you've got all these advantages, but you still have that element of making it kind of, you know, your element of secrecy is completely gone at that point. So do you think that the trend moving forward is going to be that more companies are going to be looking to go open source or do you think that we're still at a point where a lot of companies are gonna be shying away from that just because of all those concerns about intellectual property?
Jon Brennan-Badal:
I think that there is maybe a spicier thing that I'll say is that given that, I would argue that most companies within the life science industry operate under the model of charge a high price to sell to a small number of people. That type of model lends itself towards having strong IP and making it very difficult for others to kind of replicate what you're doing and replicate that high price that you charge, right. And if you open source it well, you kind of let some of that kind of secret sauce out and so I have not seen, you know, significant adoption of open source standards by many of our competitors or other companies within the life science space. And what my kind of view is, is that there will be, I think other companies that take that kind of aggressive kind of customer centric community centric view and want to maximize that and can build effective business models around charging a lot of people a small amount of money. And so I think as people figure out how to build successful businesses out of that model, you'll see a growing number of kind of open source APIs. But I think at the very least, I think you'll see at least a trend towards building more robust public facing API's as kind of an intermediate step towards open sourcing. And I think those that have really a robust adoption of those public closed source APIs may then make take the plunge, right.
Hannah Rosen:
I mean, do you have anything else, we're almost out of time here, but I just, you know, you have such a unique perspective. I'm curious, you know, do you have any other thoughts that you would like to lend to researchers maybe out there who are listening and are maybe a little bit hesitant to jump into this, you know, area of open source or, you know, your system as a whole?
Jon Brennan-Badal:
Yeah, totally like and so, you know, on that kind of, you know, to build upon that broader statement around if we really want to as a society and as a life scientist, we solve some of the most important problems over the next, you know, decade, I think now is really an important time to be really thinking about how to 1, leverage some of these important new technology trends like open source APIs or even just, you know, automation at a, you know, at a very kind of, you know, basic level. You know, so when you look at the life science industry, there's about 200,000 robots in use worldwide that have been, you know, sold in active use across kind of all the companies kind of in this space. There's about four million life scientists, lab technicians, both stocks, etcetera, etcetera worldwide that work actively day-to-day in a wet lab, which is to say that there's 20 people for every robot.
I give kind of the example of Amazon where I had sold my last company to their newest fulfillment center. So like their warehouses have literally 10 robots for every person that work in them, right, so there's a 200 fold difference of adoption of warehouses and the cutting edge. New warehouses that Amazon has versus in the life science and so when we kind of think about like hey, realistically, to solve some of these important problems, we need like 100X, 1000X more data being generated. I think we have to look really kind of long and hard around like we need to be more broadly adopting some of these important technology trends that we're seeing in other industries. So life science isn't left behind. It would be such a huge shame for important diseases not to be cured and, you know, so on and so forth because we as an industry didn't figure out how to enable scientists to be 10 times more efficient because. They're able to run 10 times as much, you know, lab work because there's 10 times as many robots. And the reason we weren't able to do that is because we weren't able to make them affordable enough, easy to use enough and we weren't able to leverage important new technology tends broadly by not, you know, leveraging open APIs and, you know, and otherwise. And so that that would be kind of my that would be my kind of key kind of parting thought, that I think it's not just, I think, something that should be a, you know, and just an interest or curiosity to check this stuff out. But I think there's actually a really important existential like almost moral or ethical kind of obligation to really be thinking about how can we be leveraging some of these important technologies to you better enable life science?
Hannah Rosen:
A lot to think about, that's for sure. Well, Jon, thank you so much for joining me today. I mean this has just been such an interesting conversation and, you know, I am really looking forward to seeing what you guys at Opentrons do and and how this open source kind of can can take us to the next level in laboratory automation.
Jon Brennan-Badal:
It was a really pleasure chatting. With you, so thank you for your time.