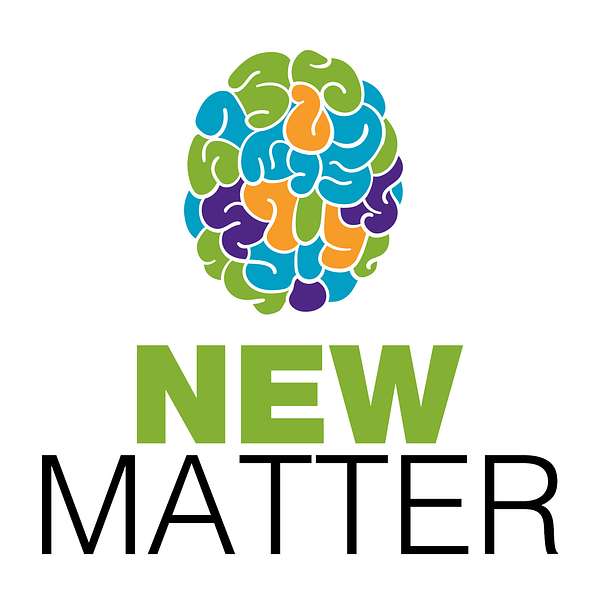
New Matter: Inside the Minds of SLAS Scientists
The official Society for Laboratory Automation and Screening (SLAS) podcast explores advances in automation, cellular imaging, big data and what's coming in the spaces between traditional scientific disciplines. Guests often include members of SLAS along with innovators, leading experts and other members of the global scientific community to highlight technology and even career stories. Episodes are released every week and subscribe to New Matter - available on all podcast players.
New Matter: Inside the Minds of SLAS Scientists
Ontology and Knowledge Graphs in Life Sciences Research | with Stephen Kahmann, M.S., and Cameron Gibbs, Ph.D.
We've invited Crown Point Technologies Co-Founder, Stephen Kahmann, M.S., and Ontologist, Knowledge Graph Engineer, Cameron Gibbs, Ph.D., to explain how ontologies and knowledge graphs are used in life sciences research.
Key Learning Points
- What ontology and knowledge graphs are
- How the data from ontologies and knowledge graphs are applied to life sciences
- Why these tools are becoming more popular in life science research
- How to use ontology and knowledge graphs in your own research
- The benefits of using these tools
Stay connected with SLAS
About SLAS
SLAS (Society for Laboratory Automation and Screening) is an international professional society of academic, industry and government life sciences researchers and the developers and providers of laboratory automation technology. The SLAS mission is to bring together researchers in academia, industry and government to advance life sciences discovery and technology via education, knowledge exchange and global community building. For more information about SLAS, visit www.slas.org.
Upcoming SLAS Events:
SLAS Europe 2025 Conference and Exhibition
- 20-22 May 2025
- Hamburg, Germany
Hannah Rosen: Hello everyone and welcome to New Matter, the SLAS podcast were we interview life science luminaries. I'm your host, Hannah Rosen, and joining me today is Steven Coleman and Cameron Gibbs of Crown Point Technologies, and we will be discussing ontologies and knowledge graphs and how they can be used in life science. Welcome to the podcast, Steve and Cameron.
Stephen Coleman: Hi, thanks, Hannah, happy to be here.
Cameron Gibbs: Yeah. Thanks for having us.
Hannah Rosen: Our pleasure! So, to start off with, I'd love it if you each could just give us a brief description of your professional background and your individual expertise.
Stephen Coleman: Sure. Yeah, I'll go ahead and start. So, you know, I've been working in the software and data management consulting space for about 12 years now, with really a specific focus on knowledge graph and ontology technologies. I actually started off as a software engineer, building tools and applications for data management. Through the years, that sort of migrated to architecture and sales. And most of that time, really, has been focused on really delivering enterprise data management solutions for customers and, you know, these spaces have been in things like manufacturing, life sciences, defense. So, you know, across these sort of big, regulated type industries, I started Crown Point technologies about a year and a half ago with the vision of delivering these advanced data management technologies to our clients.
Hannah Rosen: Great! Thanks Stephen!
Cameron Gibbs: So, I started my career in academia, I was researching knowledge representation and how it can apply to ontologies and knowledge graphs. A few years ago, I decided to take what I learned to the private sector and move into there. I've... I've worked specifically in the biomedical space, and then as well in the consulting space for the broader array of different clients, much of the same domains that Steven just mentioned. Really developing and deploying ontologies and knowledge graphs.
Hannah Rosen: So, you... you know, you kind of mentioned it a little bit Steven in your introduction, but can you give us a little bit more in depth into, you know, what is Crown Point technologies?
Stephen Coleman: Sure, yeah, I mean, we're... we're a small consulting and professional services organization. Like I mentioned, we just started about a year and a half ago. So, relatively new on the scene. But, you know, our background has been in the data management and consulting space for... for a while now, for... for, like I said, over a decade, yeah, we really wanted to start a company where we were purely focused on delivering some of these key technologies that we have sort of a strong expertise in knowledge graphs and ontologies to our customers in a lot of the same areas we've been working in... in a while... for a while now, manufacturing life sciences since those big industries. And, you know, now about a year and a half in, we're supporting customers across the US and Europe, helping them to evaluate technologies, implement data management technologies, and also look into these new approaches with knowledge graphs and ontologies.
Hannah Rosen: So, now I think the big question, because I don't know about our listeners, but I've never even heard of an ontology or a knowledge graph until I spoke to you guys, so I'm hoping that maybe, you know, we keep throwing these words around, can you guys just kind of give us a rundown of what is an ontology and what is a knowledge graph?
Cameron Gibbs: So, a typical way of thinking about an ontology is a computer readable way to represent any kind of domains, terminology, relationships and rules that might govern that vocabulary. So, I think of this as operating at a... at a layer of abstraction above the data itself. So, this is really more of a knowledge model, capturing the terminology and the schema as you used to describe and classify the data rather than just a simple data model itself. Coming to knowledge graphs, these are really a very common approach to how you could apply the ontology to your specific use case. So, you first were thinking of storing data as a graph. This would be an alternative to something more traditional like in a table or something like that. In this approach, you really think of the data as coming in nodes and edges forming in networks. So, there are the edges are relationships between whatever kind of objects you're capturing data about, and this gives you a very different kind of structure. It works really well in some... a wide array of use cases we'll talk about, I think a little bit later, and... and it helps you to ease some challenges that traditional techniques sometimes face. A good example, almost everyone's first exposure to this kind of structure is social media. So, you have a social media profile, it's connected to other profiles. Those profiles are connected to other profiles. Pretty soon you get a network, and it's very natural to think of this in terms of the relationships between the objects rather than just a table of... of classified, you know, one person all their connections, the next person, all of their connections, and then a knowledge graph itself, it's something of a buzzword, but in our world this is really just aligning a set of graph data with an ontology so you can use that... that knowledge that you've modeled in a computer readable way to really inform your data to make it understandable, accessible, integrated and... and those kinds of use cases.
Stephen Coleman: And, you know, a lot of times, we think of knowledge graphs and ontology alongside that, really as sort of an overlay technology. So, a lot of the organizations we're working with or labs that are working with data, they already have data systems that are in place, data architectures, and what they really need help with is organizing that data, bringing it together. And so the knowledge graphs set of technologies that we deploy with those really sit them on top of, or alongside the data technologies they're already using and just helps them bring that together, understand it, and get more value out of it.
Hannah Rosen: I see. So, it's basically a... would a good way to sum it up be that, like, the ontology is the way that you're categorizing the data and showing the connections between everything and the network, and then the knowledge graph is actually applying that system with the actual data you have?
Stephen Coleman: Yeah, that... that's a great way to summarize it.
Hannah Rosen: Awesome. So how can then these ontologies and knowledge graphs be used in life science research specifically?
Stephen Coleman: That's a great question. So, you know, we've... we've applied these technologies across really a wide array of use cases within life sciences. A lot of our work has been in the pharmaceutical industry. So, anything from, like, competitive intelligence research and development, clinical trial management analysis, regulatory compliance, those are all great use cases for this technology. This sort of really two sort of killer apps, so to speak, for... for knowledge graphs and ontologies, and those come down to data standardization and data integration. You know, we talked about data standardization, you know, it's really important for these regulated industries, like pharmaceuticals, for example. You think of all the compliance and reporting that goes into clinical trials, drug identification, submission, ontologies help our customers to really organize their data, organize their data systems, and be able to adhere to these regulatory guidelines and practices. That's, you know, a good example of this actually is with drug submissions. There's a standard called ISO IDP, which is identification for medicinal products. This is a big... a big standard that's coming up in the pharma industry here, and we're working with standards groups to define and promote adoption of the standard ontologies in that area. So, regulatory and compliance and standardization are really important. The second piece, really, data integration is really focused on bringing data together for analysis. And I mentioned this, I think, one of the previous questions a little bit here, but, you know, this is to help support queries, visualization advanced analytics like AI and machine learning. And what we've found, which may not be particularly surprising, that many organizations have data scattered across different data systems, different data formats, files and databases, they really struggle with collecting data to do analysis. There's been a large amount of time simply copying and pasting data, going to different systems and trying to stitch the data together, and so we want to help them automate that, help them bring the data together, and we want to be able to do that in a way that's based on open standards. And it's extensible and it aligns well with their business understanding of data. That's a really key point about, you know, applying ontologies and they really help us to do that. They provide an interoperable way to model that data and bring that data together. Or integration in life science research, clinical trial management, drug discovery, we've applied it in... in those places a lot, but one... one area that we've really focused on recently has been around smart manufacturing in the life sciences space.
So, you know, a lot of people in the industry call this... or helps them recognize sort of this idea of digital thread. So, being able to contextualize and link all of your data across development and manufacturing through these complex processes and data systems that you have and today we're working with, for example, NIIMBL, which is the National Institute for Innovation and Manufacturing Biopharmaceuticals, and pharmaceutical companies and equipment manufacturers that are industry members of that group to standardize ontologies for digital threat and biopharmaceutical manufacturing.
Cameron Gibbs: And I would like to add there's some unique technical benefits within the space of data integration, standardization, ontologies and knowledge graphs can really excel at. So, one problem often that you have when your data is distributed is, it's about the same topic or it's about the same kinds of objects. Maybe in life science is the same material, the same chemical, or biological process. But when collected in different areas, it can be a real challenge to bring that together, and that's something that these technologies can really be quite good at. So, you might have, as an example, bioreactor in a manufacturing process. You're capturing some online data from, and you're also having a sample in a test lab. It could be the same processor testing it could be these could be both coming from the same original material or cell line or something like that. And these kinds of tools can help you to bring that together to see what they have in common and... and how you can relate. Maybe there's a some kind of parameter you want to track in the... in the lab setting that you also want to keep track of in the manufacturing setting. Additionally, one of the ways these tools really kind of gone on the map was in terms of validating and reasoning over your data. So, you might want to know things, for example, when you're testing for a certain parameter on a cell line, if it crosses a certain threshold, then you... you won't want to use that cell line anymore. You could do that manually, but in... in a knowledge graph setting, if you have integrated your data and you've defined this rule in your ontology, you can flag that automatically within your... within your data set and improve, you know, your own decision-making processes. You can inform adaptive control, and there are certainly other tools that can do the job, but they often are not quite as interoperable or reusable as this because we... we can really take this holistic approach. We can define your things at this abstract level in terms of your business or... or... or scientific knowledge. Now you can apply it to the data in a really wide, interoperable way.
Hannah Rosen: So, in thinking about using these ontologies and knowledge graphs for, you know, integrating your data, analyzing your data, I'm wondering, how does one go about creating these ontologies and knowledge graphs? And, you know, can this process be automated? Because it sounds like, you know, it goes really well with large amounts of data and... and automating your data collection or processing, but I'm just having a hard time picturing, you know, how... how exactly does this whole process work?
Cameron Gibbs: Yeah, at Crown Point we have a knowledge modeling process where we... we deploy kind of step-by-step process where we work with subject matter experts and potential stakeholders that could be the same group of people in many cases. So first you really want to define a use case. You want to define something that's... it's high value, but you don't want to boil the ocean in one. We don't want to try to do everything all at the same time. So, we start out with a... a well-defined valuable use case, and we use that to kind of inform the scope. So what... what processes do we need to keep track of our measurements or... or devices that we need to keep track of with that scope in mind? Then we can really start doing the kind of knowledge modeling process. So, collecting... what terms do we need to know about? Like, how are the researchers thinking about their data and classifying their data? How are they defining these terms? Are they arranging them into a kind of hierarchy, which is... is, you know, common? They're... we might group some certain kinds of measurements together, and so there's a broad class that... that groups, those... those measurements together, and there might be other kinds of relationships we've talked about. Maybe the... a cell line can be deployed over... over a series of time and in a series of stages. So then a step-by-step relationship process, we gather all this material together and then formalize it like I mentioned before in a computer readable way. So, there's a common language that many folks in the industry use, a web ontology language or something called OWL. Once this is formalized, then we can really go to work at ingesting data into the graph structure and aligning it to that ontology. So, you really need a... a step-by-step process and... and well defined use case and scope to get started with. So, it doesn't seem so intimidating like you have to look at all your data all at the same time.
Hannah Rosen: This is kind of, the way you're describing it, it reminds me a little bit of like, knowledge trees that are so often used in machine learning. Are they... are they related or are they similar in their use?
Stephen Coleman: Yeah, I mean, I think, well you mentioned, you know, trees and... and really that's... so when we look at ontologies, they're really just a... a hierarchy of terms, right? They're the tree structure of terms that have properties and metadata, but we're also focused on relationships, so, how are these terms, which represent concepts or ideas. But then, you know, whatever domain you're working on, your lab setting or... or what have you, and how are they actually connected, right? And we... we look at that really independent of the data systems that you're working with, right? You might have several different databases that you're working with, different analytical processes or in things like machine learning. But we look at it and we say, you know, what are the types of data you're working with? Do we structure that into a hierarchy of terms? How do we create the relationships between them and then we formalize that using a standard language which is web ontology language for describing that knowledge, and that's what forms the ontology. And there's... there's tools for doing this. Tools for designing the ontologies, tools for creating these ontologies, editors and things like that. And then when we go to apply them in knowledge graphs, there are tools and technologies, platforms out there for building knowledge graphs and plotting those as well. And, you know, there's... there's open source, there's commercial, that's something that's really matured a lot in the last... last several years. So, but those are, you know, really how we apply the ontology and knowledge testing.
Hannah Rosen: Yeah, that's very interesting. Is it difficult then to integrate this, you know, unique language for the ontologies? Does it, you know, communicate, so to speak, well, with all of the other languages that people might be using to collect their data?
Stephen Coleman: It is a little bit different. The ontologies and the way we work if it is based off of a standard called RDF, which stands for Resource Description Framework. It is a specific specification from the W3C, which is a a standards body for... for defining standards around this area and other web-based standards. Most data in labs and... and enterprise settings are not using RDFS or... or web ontology language. And there is, you know, there... there are activities that we need to implement to sort of map data into those models. But when we think about how we designed these graphs and how we designed these ontologies. We're building these models in a way that... that really connects with the end user, the researcher or with the business users we're actually defining the data models within these ontologies based off of how they think about their data, how they work with their data, and a lot of times they're not the ones actually, working with the data systems, right? So, you need to actually look at the data systems, look at what you're getting from the, what we call the subject matter experts. And there needs to be a sort of mapping in between those two. And there's tooling and automation for doing that. There is an element of sort of manual processes, sort of human in the loop type of activities there. And that mapping is where you define your business rules and... and how the data really comes together to form this connected graph.
Cameron Gibbs: Yeah, I guess I would just add that, certainly an engineer will have to be involved in the loop, but the part of the nature of a kind of ontology model is it... because it works at this layer of abstraction beyond the... the data itself. It's in... in many ways more general, and it's a matter of, kind of capturing what the... what the data scientist or the researcher is... is classifying. And then... and then being able to represent it within our... our own model. In some ways it's... we are starting from something general and need to narrow our way down. And of course, there are lots of tools that... that can speed this process up and... and the engineer really overseas to make sure that mapping is happening... happening accurately.
Hannah Rosen: So, is this the sort of thing that someone could, you know, go on YouTube or find, you know, maybe like a little short course to take and kinda teach themselves and learn how to do on their own, or is this something that really requires some specialist training to learn how to implement?
Stephen Coleman: Yeah, I think, you know, as we mentioned before, these approaches are really based off of open standards, so these open standards have been around for a while. They're actually quite... well, there's a lot of tools and... and materials out there built around them, so there's a lot of material online that... that you can go find their YouTube videos. There are training courses, there are books written about these technology. These... and universities are actually now offering classes in these technologies last several years, so, you know, you don't need to be a specialist to be able to come in and do this. You do need to have sort of a... a passion for working with data, right? I mean, this is all focus on data and understanding data, modeling it and... and... and using it. You know, a lot of times we hire engineers that... that, you know, have a background in more traditional data management technologies and they sort of picked us up as... as we train them and as, you know, as they work with our customers. So, it... it doesn't require the specialist training and with a little bit of research and... and study, I think, you know, anyone could really pick up these technologies.
Cameron Gibbs: The tools have really become much more accessible in recent years as well, that... once upon a time, it was really a, almost an academic pursuit in... in how difficult some the tools could be to use but it... but there is a... a... a large number of both open source and proprietary tools that are much more user friendly, and many that are wedded to design for very particular domains. So, a life sciences researcher can go out and find actually a lot of material that's... that's ontology oriented, but very specific to what their... their interests are going to be in.
Stephen Coleman: Yeah. In fact, in the life sciences space, you know, there's a lot of existing ontologies in that space. I think, you know, life sciences were really an early adopter in these technologies, and these technologies came out about 20, 25 years ago. They've been around a little while, right? But I think they're just now picking up steam or just now really getting adopted. So, there's a lot of ontologies focused on biological concepts, you know, genes and medical codes and... and things like that. I think, you know, if you're a life science researcher and you want to get started in this space, so, if you do a little bit of searching on, you know, ontologies in your area of study or the domain you're working in, I think, more often than not, you will find something that has already been done in that space or some, you know, community group that's already doing work to build ontologies in this space. And again, getting back to that, the fact that this is really focused on standardization, that's, you know, there's a lot of work going on to try to standardize these areas and life sciences to create interoperability between groups. And so, I think... I think you'll find a lot there.
Hannah Rosen: And it actually leads perfectly into my next question, which was, I was, you know, wondering about, you know, you're saying that a lot of these... the tools, the... the language, it's all a lot... very open source. What about the specific ontologies and knowledge graphs themselves that are created maybe by individual researchers, are they typically proprietary, or are they often shared publicly for others to either use or build off of?
Cameron Gibbs: Yeah, you'll find both flavors. So, there are certainly publicly available ontologies, some that are, you know, reached the level of the standards, some from being very, very general, like the basic formal ontology, the kind of the most general way to structure your data. You can find more specific ones. The gene ontology is very popular. One, of course modeling genes and a bio portal in general is a... a great way to look at some of the publicly put out ontologies out there. In general, it's best practice to start with something that's standard and then customize as you go. Of course, in... in competitive environments, as you build your ontology out, you'll likely want it to be proprietary and will treat it as... as a kind of intellectual property, like an asset of... of any other kind. But you know, in the space there are certainly a lot of collaborative work and... and there's a really large amount of... of publicly available stuff to get you started.
Hannah Rosen: Well, and that's great. I mean, I feel like that is kind of following the trend of this sort of drug discovery and life sciences research, where it used to be, everything was kept so close to the vest. And now it seems like we're starting to finally get into an era of, you know, maybe we should actually be talking to each other and sharing what we know so that we can build off of it.
Stephen Coleman: Yeah, and I think what people are finding, you know, is... is that if you are working together as a community on data standardization, and not only does it help promote interoperability and data sharing, in some cases, there's a lot of proprietary data out there that people don't want to share. But you know, what our clients are seeing, I think, is that there's a lot of cost savings in adopting these community developed projects, right? It's sort of, you look at open-source software, it's sort of the same way, right? Everybody used to build their own software, now everybody’s using open-source components in their software, and you save a lot of time and effort doing that. And so, you know, we've seen a lot of people starting to adopt these open standards, supporting the open standards, I mean. We... we work with a couple of community groups mentioned, one called NIIMBL, which is in the biopharma space. We're working with the Pistoia Alliance, which is doing work in IBM P and in vitro pharmacology. And the members for these groups and all the big pharmaceutical companies, right, so they're all invested and investing in trying to create standard ontologies and approaches to managing this data, because they know that if they if they do that, they're going to see a lot of cost savings and internally with how they work with their data.
Hannah Rosen: Yeah, definitely. Are there any research situations where maybe an ontology or knowledge graph would not be appropriate to use? And in that case, are there other ways of organizing data that people should be using instead?
Cameron Gibbs: So, there's... there's certainly areas where ontologies and knowledge graphs are not going to apply particularly well, so many of the cases we've been talking about so far are much more analytical oriented use cases. You want to bring your data together, you want to better understand it, apply some kind of analysis to it, might have really, highly transactional sort of data that's constantly being updated all the time. E-commerce data can be like this in a scientific context. You might have time series data. So, think like a sensor that's continuously updating the new data that you're collecting that might not be the most appropriate way to... to apply a knowledge graph. Now you could have applications where you might want to be recording information about the sensor. You might, say, be able to register that you're collecting a certain kind of data that might be involving a certain kind of measurement process, or involving certain kinds of devices, and that could make more accessible. The sensor data itself, which might live outside of the knowledge graph in some way, so you can have this external data system continuously being updated, but the knowledge graph will make that more accessible and in some ways more understandable.
Stephen Coleman: We look at those types of solutions as sort of metadata solutions, right? So, you're sort of managing metadata about all of these other data elements that you're collecting. So even if you don't want to store all that data in the graphs, because maybe it's too much data, or maybe there's just not a lot of richness to the data in the data model. You just want to be able to point to, sit and talk about the type of data that's there, what it's related to, and when we look at knowledge graphs, you know, those relationships are really what's important, right? So, helping you find that data helping you get access to it, helping you reuse it, this is all the FAIR principles and then, you know, just... just making that more accessible to your enterprise, even if you're not storing all that data in the graph. There's other types of techniques that, you know, knowledge graphs don't replace or don't, you know, solve these problems. Your areas of like, your advanced analytics like machine learning, artificial intelligence, natural language processing is a good example. You know, knowledge graphs and ontologies still solve those problems that they're... they're sort of different things, but applying knowledge graphs miss those analytical techniques can really help improve those solutions. Organizing and normalizing the data to feed into those analytical processes and... or storing the results of those processes back into the... for connecting with other data sources, those are really good use cases as well.
Hannah Rosen: That’s really interesting. So it's more like, you know, ontologies and knowledge graphs, they’re not replacements to machine learning and AI. And AI is more... but they're complementary. They help to improve your ability to use machine learning and AI.
Cameron Gibbs: I'll just add within this area of machine learning, AI, natural language processing, one of the really exciting areas of research going on is... is how knowledge graphs can work together with those kinds of technologies. So, machine learning autograph has become, you know, one kind of exciting area of research. I think that people are just beginning to see the potential applications of that even within our projects where we're exploring the possibilities of that, that we can use these kinds of tools.
Hannah Rosen: Well, so it seems like you might be able to use a knowledge graph to train a machine learning model potentially.
Stephen Coleman: Yeah, yeah, the knowledge graph itself will do the training, but you can, you know, generate training data sets. You can provide that and you can improve the effectiveness of the training process itself. So, there's actually a lot of research going into that. There's also, you know, some... some techniques that are sort of new in that area, things like explainable AI, for example. You know, the richness that goes into ontologies and knowledge graphs supporting explainable AI to explain why certain decisions are made by your... by your models and really what we get back to you and these types of advanced analytics solutions really need a strong data foundation, and that's what the knowledge graph and ontology provides to really support those.
Hannah Rosen: Yeah, it sounds like there's a ton of potential that we're just starting to kind of really tap into, yeah.
Stephen Coleman: Yeah, absolutely.
Hannah Rosen: So, if there's a researcher out there who's interested in starting to use these ontologies and knowledge graphs, you know, what are some of the first steps that they should take to integrate this into their work?
Cameron Gibbs: Well, there's always the temptation. Speaking of, you know, personal experience that you see a cool new tool and you just want to use it as much as you can, and we definitely recommend, resist that temptation. You want to have, I mean, a particular use case that you can apply it to. You don't... as... as we talked about earlier, you don't have to look at all data that your organization is collecting and... and figuring out how to bring it together. Think about what's a particular high value use case you can get if you can bring some of that data together and... and you can get a better understanding of some of that data and have a more limited scope. Part of the nature of these kinds of models is that they can be built up over time. It doesn't break the model to add more to it. It... it... it... it's not as though you have to set all the foundations in place and you... and you can't really move it at all. So, you can apply an iterative approach, expand your scope, expand your use cases from there. And I would just recommend people would... would look online, see what's out theres and in many of your particular application areas, there's probably work already going on, professional organizations, you're... you're part of. You might... you might be surprised to find that... that some of your colleagues are already exploring these options and... and they've gotten... gotten the research started already in figuring out how to apply.
Stephen Coleman: Yeah, that... that's a great way to describe it. I mean, that target is... use case driven approach is definitely the way to start. Of course, you can always give us a call too. We'd be happy to help you guys get started.
Hannah Rosen: Ohh I'm sure. Well Steven, Cameron, thank you guys so much for joining me today. I've learned a ton and I feel like next time somebody comes up to me and says the word ontology I will know what the heck, they're talking about, which is always thrilling for me. And for any of you listeners, if you're interested in learning more, I'm sure you can reach out to Steven and Cameron at Crown Point Technologies. They'd be happy to talk more and help you get started.
Stephen Coleman: Thanks, Hannah. Thanks for having us, really enjoyed being here.
Cameron Gibbs: Yeah. Thanks so much, it was a pleasure.